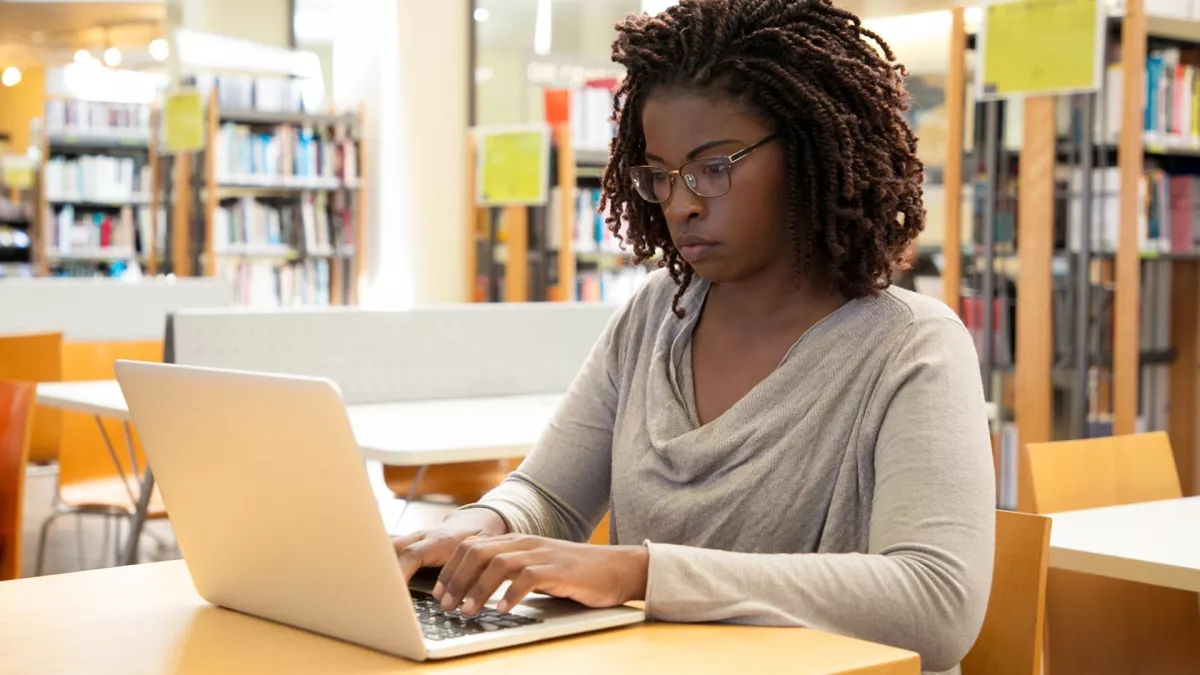
V. Five Principles for the Use of Artificial Intelligence in Education
Introduction
The Task Force on Artificial Intelligence has proposed a Policy Statement to guide the NEA’s work to advocate for the equitable, ethical, and evidence-supported development and implementation of AI technologies to benefit all students and educators. This proposed Policy Statement is in response to the recent emergence of AI in teaching and learning while also building on policies and actions the NEA has taken in the past to safeguard students, educators, and public schools. As students, educators, schools, and campuses begin to adopt AI, it is imperative that they do so in ways that maximize benefits and minimize or eliminate harms. To this end, the Task Force offers five guiding principles in the proposed Policy Statement that provide a framework for the NEA’s advocacy, policy, and practice work in this area.
A. Principle 1: Students and educators must remain at the center of education
1. Text of the Principle
Learning happens, and knowledge is constructed through social engagement and collaboration, making interpersonal interaction between students and educators irreplaceable. Cecilia Ka Yuk Chan and Louisa H. Y. Tsi, "The AI Revolution in Education: Will AI Replace or Assist Teachers in Higher Education?," arXiv: 2305.01185 (2023), http://arxiv.org/pdf/2305.01185; Cathy McKay and Grace Macomber, "The Importance of Relationships in Education: Reflections of Current Educators," Journal of Education 203, no. 4 (2021), https://doi.org/10.1177/00220574211057044; National Academies of Sciences, Engineering, and Medicine, How People Learn II: Learners, Contexts, and Cultures (2018), https://doi.org/10.17226/24783. Go to reference The use of AI should not displace or impair the connection between students and educators, a connection that is essential to fostering academic success, critical thinking, interpersonal and social skills, emotional well-being, creativity, and the ability to fully participate in society. AI-enhanced tools that undermine any of these critical aspects of teaching and learning should not be employed.
We envision AI-enhanced technology as an aid to public educators and education, not as a replacement for meaningful and necessary human connection. To move AI forward as an additive resource and tool, professionally and socially diverse educators (across race/ethnicity, gender, disability status, positions, and institutional levels) must be included in decision-making – inclusive of AI vetting, adoption, deployment, and ongoing use – to guarantee that these tools are used to improve job quality and enhance performance.
AI technology tends to reflect the perspectives—and biases—of the people who develop it. Furthermore, developers may not notice when their tools are biased against or do not adequately reflect the needs of people who differ from them demographically or in other ways. Notably, technology developers are overwhelmingly younger, White, cisgender, heterosexual, male, and people without disabilities. Stack Overflow, 2022 Developer Survey (2022), https://survey.stackoverflow.co/2022/. Go to reference Actively involving a diverse and intersectional array of educators, including those with disabilities, in the development, design, and evaluation of AI systems ensures technology that is not only compliant with accessibility standards but also genuinely user-centric. Including the diverse and intersectional perspectives and experiences of people who are Native, Asian, Black, Latin(o/a/x), Middle Eastern and North African, Multiracial, and Pacific Islander, LGBTQ+, and from all economic backgrounds and abilities is essential if this technology is to be effective in its educational purpose.
Artificial intelligence should not be used to undercut educators by exposing them to unnecessary surveillance, undermining their rights, or taking over core job functions that are best done by humans. These tenets should be reflected in and protected through collective bargaining, labor-management collaboration, and state laws.
AI-informed analyses and data alone should never be used for high-stakes or determinative decisions. While such data might be included among several other factors, the degree of its importance, weight, and reliability must be carefully considered in matters concerning items such as, but not limited to: employee evaluations; student assessment, placement, graduation, and matriculation; disciplinary matters; diagnoses of any kind; and matters of safety and surveillance. These decisions must rely primarily on the professional expertise and judgment of humans, who must consider equity, diversity, access, human rights, and other appropriate contextual considerations. See also, NEA’s Policy Statement on Teacher Evaluation and Accountability. Go to reference
2. Connections to Existing NEA Policies
This principle closely relates to the NEA’s Policy Statement on Digital Learning. Specifically, the existing Policy Statement identifies technology as a tool used to enhance and enrich instruction for students and states that it should not be used to replace education employees who work with students or limit their employment. This statement also recognizes that student learning needs are best met by public school districts and postsecondary institutions working in collaboration with educators and local associations to develop comprehensive and thorough digital learning plans to address all the elements of incorporating technology into teaching and learning.
The proposed Policy Statement also relates to Resolution B-66: Technology in the Educational Process, which states that education employees, including representatives of the local affiliate, must be involved in all aspects of technology utilization, including planning, materials selection, implementation, and evaluation. Additionally, the Resolution states that the impact of technology on education employees should be subject to local collective bargaining agreements. Lastly, Resolution E-6: Development of Materials states that public school teachers and postsecondary faculty should be involved in the development and field testing of all educational materials offered for adoption or purchase by public school districts and education institutions. The Task Force believes that the same standards outlined in these existing NEA policies should be applied to AI technologies to prioritize a human-centered educator workforce.
3. Background Research and Information
The foundation of student learning is built on the relationships that thrive in human-centered schools. National Academies of Sciences, How People Learn II: Learners, Contexts, and Cultures. Go to reference Learning happens, and knowledge is constructed through social-emotional engagement and collaboration, making human interaction among educators and students irreplaceable. Chan and Tsi, “The AI Revolution in Education: Will AI Replace or Assist Teachers in Higher Education?”; McKay and Macomber, “The Importance of Relationships in Education: Reflections of Current Educators.” Go to reference Human educators possess unique qualities—such as critical thinking, creativity, and emotions—that cannot be sufficiently recreated by AI tools. Lauraine Langreo, "6 Things Teachers Do That AI Just Can't," Education Week, Sept. 7, 2023, https://www.edweek.org/technology/6-things-teachers-do-that-ai-just-cant/2023/09. Go to reference Educators inspire and help students in thousands of unseen ways and understand learners within the context of the classroom, the school, and the community in a manner that computers never will. The foundation for this humanistic side of teaching is building and maintaining strong relationships that are grounded in mutual respect, trust, and empathy.
Relationships are more than just knowing the student’s names; they encompass mutual respect, building trust, and feelings of safety. Relationships can make or break a student’s experience at school; in fact, student success hinges on a teacher’s ability to build effective relationships with students... students’ sense of support (e.g., being liked, respected, and valued by the teacher) predicts their expectancies for success and valuing of subject matter. McKay and Macomber, "The Importance of Relationships in Education: Reflections of Current Educators." Go to reference
Education also goes well beyond acquiring content knowledge—schools are where students learn to collaborate, think creatively and critically, and be fully engaged members of society. "The OECD Learning Compass 2030," OECD, 2024, https://www.oecd.org/education/2030-project/teaching-and-learning/learning/; "Education GPS – OECD: Social & Health Outcomes," OECD, 2024, https://gpseducation.oecd.org/revieweducationpolicies/#!node=41767&filter=all. Go to reference Furthermore, educators and schools are fundamental to the social safety net in terms of responding to the needs of the whole child. Emily Kaplan, "Unfairly, Schools and Teachers Are America’s Anti-Poverty Safety Net," Edutopia (May 5, 2022). https://www.edutopia.org/article/unfairly-schools-and-teachers-are-americas-anti-poverty-safety-net/; Karina Piser, "How Public Schools Became America’s Social Safety Net," The Nation, February 19, 2021, https://www.thenation.com/article/society/community-schools-coronavirus/. Go to reference Thus, while artificial intelligence can aid educators, it can never replace them. Equitable and effective education can only happen when human interactions are at the center of the learning experience.
When implementing AI, it is paramount that human relationships remain at the forefront, leveraging educational technology to enhance and augment rather than replace the human interactions and relationships that are fundamental to effective education for all students. Unfortunately, given the alarming pre-K–12 educator shortage, many districts are looking for ways to increase staffing efficiencies across all positions, including the use of AI tools. Rachel Post, "How Can AI Help Solve Teacher Shortages?," AASPA Blog, February 1, 2024, https://www.aaspa.org/news/how-can-ai-help-solve-teacher-shortages. Go to reference Faculty, staff, and graduate student positions also face challenges from AI. Chan and Tsi, "The AI Revolution in Education: Will AI Replace or Assist Teachers in Higher Education?." Go to reference There are well-founded fears that AI may replace or change educator jobs in significant ways. Ziyan Dong, "Research on the Impact of Artificial Intelligence on the Development of Education," Lecture Notes in Education Psychology and Public Media 28 (2023), https://doi.org/10.54254/2753-7048/28/20231364. Go to reference Policymakers, AI developers, school boards, and administrators should be held accountable for prioritizing human agency when implementing AI in education to protect students and educators.
The principle of “aid but not replace” is most central in the context of high-stakes decisions such as: employee evaluations; student assessment, placement, graduation, and matriculation; disciplinary matters; student diagnoses of any kind; and matters of school safety and surveillance. We have already seen problematic implementations of AI in determinative decision-making, including a Texas A&M University at Commerce professor who threatened to fail an entire class, preventing some students from graduating, because an AI detector had incorrectly tagged student work as AI-generated. Pranshu Verma, "A Professor Accused His Class of Using ChatGPT, Putting Diplomas in Jeopardy," The Washington Post, May 18, 2023, https://www.washingtonpost.com/technology/2023/05/18/texas-professor-threatened-fail-class-chatgpt-cheating/. Go to reference In Nevada, an AI algorithm was used to determine pre-K–12 school funding. As a result, the number of students defined as "at risk” was reduced from 288,000 in the 2022–2023 school year to only 63,000 the following year, making them ineligible for supplemental state funding. Jordan Abbott, "When Students Get Lost in the Algorithm: The Problems with Nevada's AI School Funding Experiment," New America, 2024, http://newamerica.org/education-policy/edcentral/when-students-get-lost-in-the-algorithm-the-problems-with-nevadas-ai-school-funding-experiment/. Go to reference
Concerns about the use of AI in high-stakes decisions are particularly salient for students and educators with disabilities. AI can be discriminatory and may inaccurately make assumptions and assertions about students based on their disability and other descriptive factors, leading to incorrect and biased eligibility decisions. Decisions about individualized education programs (IEP) and 504 plans should be made in an individualized manner by the designated members of the IEP team, considering students' unique strengths, needs, and services. There is also significant concern that overreliance and overconfidence in this technology could lead to students being singled out or identified as having a disability without being evaluated by a licensed and trained professional. AI should never serve as the sole diagnostic tool for any disability or replace evaluations by human professionals.
To guard against these troubling uses of AI, educators must be included in the development, selection, implementation, and assessment of AI tools in all aspects of education. President Joe Biden, in his Executive Order on the Safe, Secure, and Trustworthy Development and Use of Artificial Intelligence, succinctly summarizes the need to keep humans in the loop.
AI should not be deployed in ways that undermine rights, worsen job quality, encourage undue worker surveillance, lessen market competition, introduce new health and safety risks, or cause harmful labor-force disruptions. The critical next steps in AI development should be built on the views of workers, labor unions, educators, and employers to support responsible uses of AI that improve workers’ lives, positively augment human work, and help all people safely enjoy the gains and opportunities from technological innovation. "Executive Order on the Safe, Secure, and Trustworthy Development and Use of Artificial Intelligence," The White House, updated October 30, 2023, https://www.whitehouse.gov/briefing-room/presidential-actions/2023/10/30/executive-order-on-the-safe-secure-and-trustworthy-development-and-use-of-artificial-intelligence/. Go to reference
Along these same lines, humans must remain central when it comes to evaluating educators. As the education landscape becomes increasingly digitized, the use of AI in educator evaluations raises several concerns among educators regarding equity, accuracy, and transparency. AI’s inability to understand the contextual nuances of teaching and learning would devalue the professional expertise of human evaluators who can exercise judgment. In addition, although researchers understand the general processes of how AI output is reached, the specific process of how a model arrives at a specific output is not. Warren J. von Eschenbach, "Transparency and the Black Box Problem: Why We Do Not Trust AI," Philosophy & Technology 34, no. 4 (2021), https://doi.org/doi:10.1007/s13347-021-00477-0. Go to reference Educator evaluations that may determine employment, pay, or related considerations are too important to be left to systems that aren’t able to consider accurate human judgment or fully explain justifications for their reasoning.
Artificial intelligence should never be the sole or definitive decider in evaluations or employment decisions. Additionally, joint labor-management committees should work together to develop evaluation processes and practices that lead to collaborative conversations, useful feedback, and teacher growth. In states that allow bargaining, education unions should leverage contract language that safeguards educators from limited and harmful evaluative practices that aim to punish and demean educators. Douglas F. Warring, "Teacher Evaluations: Use or Misuse?," Universal Journal of Educational Research 3, no. 10 (2015), https://doi.org/10.13189/ujer.2015.031007. Go to reference Transparency involves dialogue and cooperation among educators, administrators, and AI experts to address issues, refine evaluation standards, and uphold ethics.
- 33 Cecilia Ka Yuk Chan and Louisa H. Y. Tsi, "The AI Revolution in Education: Will AI Replace or Assist Teachers in Higher Education?," arXiv: 2305.01185 (2023), http://arxiv.org/pdf/2305.01185; Cathy McKay and Grace Macomber, "The Importance of Relationships in Education: Reflections of Current Educators," Journal of Education 203, no. 4 (2021), https://doi.org/10.1177/00220574211057044; National Academies of Sciences, Engineering, and Medicine, How People Learn II: Learners, Contexts, and Cultures (2018), https://doi.org/10.17226/24783.
- 34 Stack Overflow, 2022 Developer Survey (2022), https://survey.stackoverflow.co/2022/.
- 35 See also, NEA’s Policy Statement on Teacher Evaluation and Accountability.
- 36 National Academies of Sciences, How People Learn II: Learners, Contexts, and Cultures.
- 37 Chan and Tsi, “The AI Revolution in Education: Will AI Replace or Assist Teachers in Higher Education?”; McKay and Macomber, “The Importance of Relationships in Education: Reflections of Current Educators.”
- 38 Lauraine Langreo, "6 Things Teachers Do That AI Just Can't," Education Week, Sept. 7, 2023, https://www.edweek.org/technology/6-things-teachers-do-that-ai-just-cant/2023/09.
- 39 McKay and Macomber, "The Importance of Relationships in Education: Reflections of Current Educators."
- 40 "The OECD Learning Compass 2030," OECD, 2024, https://www.oecd.org/education/2030-project/teaching-and-learning/learning/; "Education GPS – OECD: Social & Health Outcomes," OECD, 2024, https://gpseducation.oecd.org/revieweducationpolicies/#!node=41767&filter=all.
- 41 Emily Kaplan, "Unfairly, Schools and Teachers Are America’s Anti-Poverty Safety Net," Edutopia (May 5, 2022). https://www.edutopia.org/article/unfairly-schools-and-teachers-are-americas-anti-poverty-safety-net/; Karina Piser, "How Public Schools Became America’s Social Safety Net," The Nation, February 19, 2021, https://www.thenation.com/article/society/community-schools-coronavirus/.
- 42 Rachel Post, "How Can AI Help Solve Teacher Shortages?," AASPA Blog, February 1, 2024, https://www.aaspa.org/news/how-can-ai-help-solve-teacher-shortages.
- 43 Chan and Tsi, "The AI Revolution in Education: Will AI Replace or Assist Teachers in Higher Education?."
- 44 Ziyan Dong, "Research on the Impact of Artificial Intelligence on the Development of Education," Lecture Notes in Education Psychology and Public Media 28 (2023), https://doi.org/10.54254/2753-7048/28/20231364.
- 45 Pranshu Verma, "A Professor Accused His Class of Using ChatGPT, Putting Diplomas in Jeopardy," The Washington Post, May 18, 2023, https://www.washingtonpost.com/technology/2023/05/18/texas-professor-threatened-fail-class-chatgpt-cheating/.
- 46 Jordan Abbott, "When Students Get Lost in the Algorithm: The Problems with Nevada's AI School Funding Experiment," New America, 2024, http://newamerica.org/education-policy/edcentral/when-students-get-lost-in-the-algorithm-the-problems-with-nevadas-ai-school-funding-experiment/.
- 47 "Executive Order on the Safe, Secure, and Trustworthy Development and Use of Artificial Intelligence," The White House, updated October 30, 2023, https://www.whitehouse.gov/briefing-room/presidential-actions/2023/10/30/executive-order-on-the-safe-secure-and-trustworthy-development-and-use-of-artificial-intelligence/.
- 48 Warren J. von Eschenbach, "Transparency and the Black Box Problem: Why We Do Not Trust AI," Philosophy & Technology 34, no. 4 (2021), https://doi.org/doi:10.1007/s13347-021-00477-0.
- 49 Douglas F. Warring, "Teacher Evaluations: Use or Misuse?," Universal Journal of Educational Research 3, no. 10 (2015), https://doi.org/10.13189/ujer.2015.031007.
B. Principle 2: Evidence-based AI technology must enhance the educational experience
1. Text of the Principle
Artificial intelligence should only be adopted once there is data supporting a tool’s appropriateness and efficacy with potential users and, for instruction-focused AI, its alignment with high-quality teaching and learning standards and practices. This evidence should come either from research conducted and reviewed by independent researchers or from industry-sponsored research that adheres to the same standards of methodology and peer review as independent research. If such research is unavailable, AI may be adopted on a pilot or trial basis if the evidence is being collected and analyzed in a timely manner, with an agreement in place to cease the use of the technology if the results of the research do not show the intended benefits or do not serve educational goals.
Close attention must be paid to the needs of our most vulnerable learners, including students with disabilities, early learners, and emergent multilingual learners. AI technology must not conform to a purely ableist and privileged standard that neither serves nor adapts to the educational needs of students with disabilities. User cases that aid in the development of effective AI tools in education must be based on a range of disabilities (i.e., learning disabilities, hearing impairments, visual impairments, etc.). While some AI technology may improve accessibility and enhance these students’ educational experiences, these students are susceptible to harm if AI is used inappropriately. There must be dedicated research and the establishment of clear guidance to help our schools ensure that AI-enabled technology is effective and appropriate for these students.
It is critical that systems, processes, and structures are created to ensure intentional and ongoing attention is paid to the extent to which biases built into AI technology and uses of AI-generated data further perpetuate racial injustice and social inequities in education. AI tools need to be carefully evaluated by educators, Native communities and communities of color, and rural communities to ensure these tools reflect the diversity of students’ backgrounds and experiences and proactively avoid inequitable access to high-quality technology and internet access. We must also ensure these tools do not subject students who are Native, Asian, Black, Latin(o/a/x), Middle Eastern and North African, Multiracial, or Pacific Islander to higher surveillance than their White peers, perpetuate school-to-prison and school-to-deportation pipelines, or create an over-reliance on content and assessment delivered by AI-enhanced technology rather than that of qualified educators.
Assessment of AI efficacy must not end after a tool is adopted. Innovations in technology, pedagogy, and content are ongoing, and AI tools must be reassessed regularly by educators to ensure they continue to provide the intended benefits and have not created unanticipated problems. Educators must be involved in both the initial and ongoing assessment of AI tools so that AI is used only if it will enhance, rather than detract from, students’ educational experiences and their well-being. Educator involvement is critical to ensure that AI is implemented in ways that are effective, accurate and appropriate for learners at all levels.
2. Connections to Existing NEA Policies
AI tools and resources used for teaching and learning must be thoroughly researched. This principle aligns with existing NEA policy statements, resolutions, and legislative programs that emphasize the importance of evidence-based practices and resources. Specifically, the NEA’s Policy Statements on Safe, Just, and Equitable Schools and Community Schools emphasize the use of evidence-based practices that ensure all students’ needs are met. Resolution A-14: Financial Support of Public Education states that provisions must be made for research, development, implementation, continuation, and improvement in education practices. Resolution A-36: School Restructuring underscores evidence-based plans that address the needs of the whole child. Similarly, Resolution B-74: Social-Emotional Learning calls for evidence-based instructional methods. Lastly, Legislative Program: I.K.16, High Quality Public Education supports the promotion of research and development of knowledge, including access by students to advanced technological resources and teaching.
This principle advocates for educator involvement when researching AI tools. Resolution E-1: Instruction Excellence recommends that education employees collaborate in the research, development, and field testing of new instructional methods and materials. Likewise, Legislative Program: I.H.c.02, High Quality Public Education, Education Research and Development calls for the participation of educators in research efforts. The Task Force proposes that the same standards outlined in the above statements, resolutions, and legislative program amendments should prioritize evidence-based AI technologies that enhance the educational experience of students and educators.
3. Background Research and Information
At present, the evidence base about the use of AI is minimal but growing. For a review of research on the use of AI in K–12 contexts from 2017–2022, see: Florence Martin, Min Zhuang, and Darlene Schaefer, "Systematic Review of Research on Artificial Intelligence in K–12 Education (2017–2022)," Computers and Education: Artificial Intelligence 6 (2024), https://www.sciencedirect.com/science/article/pii/S2666920X23000747. Go to reference In Education International’s 2023 overview of the current state of AI in education, Wayne Holmes notes, “There remains little evidence that what is good for the technology industry is good for the world; similarly, there is little evidence that what is promoted by the AI industry is good for students and teachers.” Holmes, The Unintended Consequences of Artificial Intelligence and Education. Go to reference
Much of the research and evidence that is available has been generated by ed-tech companies rather than by independent researchers. Independent research is important because academic scholars hold one another to methodological standards and norms of transparency that may or may not be used in industry contexts.
That said, the Task Force acknowledges that developing an evidence base takes time, and it is both impossible and inadvisable to halt the use of AI entirely. The emergence of AI provides a fruitful opportunity for the development of research-practice partnerships through which academic researchers and educators partner on projects of mutual interest. For more on research-practice partnerships in education, see: "National Network of Education Research-Practice Partnerships," National Network of Education Research-Practice Partnerships, accessed April 4, 2024, https://nnerpp.rice.edu/. Go to reference Research-practice partnerships provide benefits to everyone involved. Developers gain insights into how their tools are used in actual schools and classrooms and receive direct feedback from end-users. Researchers are able to increase their confidence that their studies have both internal validity—that what the phenomenon they think is being captured is what is captured—and external validity—that their findings apply outside of an artificial setting created for the purposes of research. Most importantly, educators are given a voice in the development process by being able to give both formative and summative feedback on AI tools. These opportunities also provide educators with opportunities to hone their understanding of the research process. When possible, students should also be actively engaged in the research process.
Research on AI must also be sure to look at the effects of this technology on different groups of students. A tool that works for one group of students may not work for another, and differential effects might suggest algorithmic issues, such as bias. Depending on age, ability, language, background, and other factors, students may be more or less vocal about issues they encounter with AI tools, and educators and developers may be more or less willing to listen to them. Conducting research through an equity lens will help create environments in which developers and researchers obtain an accurate understanding of when and how a tool leads to the desired outcomes.
The collection and analysis of evidence must continue as long as an AI tool is in use. These tools are constantly being updated and new data introduced into them. In addition, instructional needs may change over time. Consistent, ongoing evaluation that includes the perspectives of students and educators will ensure that AI tools are providing the intended benefits without exposing anyone to undue harm.
- 50 For a review of research on the use of AI in K–12 contexts from 2017–2022, see: Florence Martin, Min Zhuang, and Darlene Schaefer, "Systematic Review of Research on Artificial Intelligence in K–12 Education (2017–2022)," Computers and Education: Artificial Intelligence 6 (2024), https://www.sciencedirect.com/science/article/pii/S2666920X23000747.
- 51 Holmes, The Unintended Consequences of Artificial Intelligence and Education.
- 52 For more on research-practice partnerships in education, see: "National Network of Education Research-Practice Partnerships," National Network of Education Research-Practice Partnerships, accessed April 4, 2024, https://nnerpp.rice.edu/.
C. Principle 3: Ethical development and use of AI technology and strong data protection practices
1. Text of the Principle
Artificial intelligence is far from flawless and requires human oversight, checks, and balances. Primary areas of concern include algorithmic bias, inaccurate or nonsensical outputs, violations of student and educator data privacy, and the considerable environmental impact of AI energy use. AI tools must be carefully vetted prior to deployment and monitored after implementation to mitigate these hazards, guarantee ongoing transparency, and confirm that tools comply with current local, state, and federal laws. States, local districts, and higher education institutions should evaluate (and strengthen where necessary) their existing data governance plans prior to adopting AI tools. Particular attention must be paid to AI tools that aim to play any role in assessing/evaluating students or educators or would have monitoring or surveillance functions. AI tools proposed for any of these purposes should be approached with caution; evaluated, understood, and agreed to by appropriate interest holders (including students, educators, and families); and used with the understanding that AI data models and programming are biased, incomplete, quickly become outdated, and can result in unreliable and harmful results, particularly for Native students, students of color, and students with disabilities.
Educators, parents, and students must be made aware of what and how AI tools are used in schools and on campuses. Educators must receive ongoing learning opportunities that enable them to identify ethical hazards and how to handle them effectively if they arise. Institutional structures, such as review boards or scheduled audits, should also be put in place to enforce high-quality standards for the use of AI. Data collected through AI should be subject to protocols providing transparency about the types of data being collected and how the data is stored, utilized, and protected. These protocols must also clearly articulate whether and to what degree AI is used for any form of monitoring or surveillance in educational settings and how this data will be governed. Additionally, these protocols must ensure the proprietary rights of students and educators in their original work.
Although these technologies operate in virtual spaces, AI and the cloud will consume increasing amounts of energy and require larger quantities of natural resources, which has the potential to increase greenhouse gas emissions. At present, generating a single image using a powerful AI model consumes as much energy as fully charging your smartphone. While it is nearly impossible for researchers to evaluate the full extent of the negative environmental impacts of AI technologies, decision-makers in school settings should be aware of the connection between AI and the environment and be mindful of environmental impacts throughout the planning and implementation phases.
2. Connections to Existing NEA Policies
This principle relates to several existing policy statements and resolutions. The Policy Statement on Digital Learning recognizes the importance of safeguarding educators' and students' personal data along with Resolution F-34: Right to Privacy for Education Employees. Moreover, the Policy Statement on Digital Learning supports educator ownership of copyrighted materials. Likewise, Resolution B-67: Fair and Equitable Access to Technology states that any documentation material produced from internet access should be properly cited and comply with copyright laws. Resolution E-10: Intellectual Property and Access to Copyrighted Materials supports educator and student proprietary rights. A number of amendments in the NEA Legislative Program express the NEA’s support for protecting student and educator data privacy, including: Legislative Program: I.E.27, High Quality Public Education, Safe Schools; Legislative Program: III.A.21, A Voice in the Workplace, Public Employee Rights; and Legislative Program: IV.B.d.05 and IV.B.d.08, Good Public Policy and Human and Civil Rights, Privacy, Freedom of Information, and Governmental Intervention.
Several existing NEA policies touch on areas of social justice, civil rights, and discrimination. All of these concepts relate to biases that can exist in AI-enabled systems and contribute to inequality, injustice, and discrimination. We relied heavily on guidance from the Policy Statement on Safe, Just, and Equitable Schools; Resolution I-55: White Supremacy Culture; Resolution B-15: Racism, Sexism, Sexual Orientation, Gender Identity, and Gender Expression Discrimination; and Resolution B-36: Education for All Students with Disabilities. The same standards outlined in the above statements and resolutions should be applied to ensure the development and implementation of ethical AI technologies in public schools.
3. Key Federal Laws
The United States does not have a comprehensive law that covers data privacy; instead, there are federal and state laws that cover various types of data privacy, such as financial data or health information. As of this writing, two states, California "California Consumer Privacy Act (CCPA)," State of California, Department of Justice, updated March 13, 2024, https://oag.ca.gov/privacy/ccpa. Go to reference and Virginia, "Code of Virginia—Chapter 53. Consumer Data Protection Act," Virginia Law, 2021, https://law.lis.virginia.gov/vacodefull/title59.1/chapter53/. Go to reference have enacted comprehensive state privacy laws.
It is imperative that policymakers and all of society learn from the mistakes made by allowing unregulated social media and unaccountable social media platforms to buy and sell our data to the highest bidder without consent. There is now mounting evidence that children who have higher exposure to social media have a greater risk of developing mental health problems, particularly adolescents. U.S. Department of Health and Human Services, Office of the U.S. Surgeon General, Social Media and Youth Mental Health (2023), https://www.hhs.gov/surgeongeneral/priorities/youth-mental-health/social-media/index.html. Go to reference
In recent years, two major federal legislative proposals surfaced, the American Privacy Rights Act Committee on Energy and Commerce, "Committee Chairs Rodgers, Cantwell Unveil Historic Draft Comprehensive Data Privacy Legislation," news release, April 7, 2024, https://energycommerce.house.gov/posts/committee-chairs-rodgers-cantwell-unveil-historic-draft-comprehensive-data-privacy-legislation. Go to reference and the American Data Privacy and Protection Act (ADPPA), "H.R.8152 – 117th Congress (2021–2022): American Data Privacy and Protection Act," U.S. House of Representatives, 2022, https://www.congress.gov/bill/117th-congress/house-bill/8152. Go to reference both aiming in different ways to address data privacy, algorithm transparency, and other concerns in a comprehensive manner. The likelihood of passage for these proposals in not known at this time; however, it is encouraging to see substantive, high-quality policy proposals circulating.
While President Biden’s 2023 Executive Order on the Safe, Secure, and Trustworthy Development and Use of Artificial Intelligence gives broad guidance and does not apply exclusively to educational environments, it does direct federal agencies, including the U.S. Department of Education. The Executive Order specifically directs the Department of Education to:
…help ensure the responsible development and deployment of AI in the education sector, the Secretary of Education shall, within 365 days of the date of this order, develop resources, policies, and guidance regarding AI. These resources shall address safe, responsible, and nondiscriminatory uses of AI in education, including the impact AI systems have on vulnerable and underserved communities, and shall be developed in consultation with stakeholders as appropriate. They shall also include the development of an “AI toolkit” for education leaders implementing recommendations from the Department of Education’s AI and the Future of Teaching and Learning report, including appropriate human review of AI decisions, designing AI systems to enhance trust and safety and align with privacy-related laws and regulations in the educational context, and developing education-specific guardrails. The White House, "Executive Order on the Safe, Secure, and Trustworthy Development and Use of Artificial Intelligence." Go to reference
Related to the data privacy of students, there are currently two federal laws that are worth mentioning.
The Family Educational Rights and Privacy Act of 1974 (FERPA) is described by the U.S. Department of Education as “a Federal law that protects the privacy of student education records. The law applies to all schools that receive funds under an applicable program of the U.S. Department of Education.” "Family Educational Rights and Privacy Act (FERPA)," U.S. Department of Education, 2021, https://www2.ed.gov/policy/gen/guid/fpco/ferpa/index.html. Go to reference
The last regulatory updates to FERPA predate the widespread use of technology in educational environments, including the storage of education records, the technological generation of records, and the use of technology to support and assess students. School districts and education institutions that are subject to FERPA must interpret this law for how data is accessed, used, and stored in light of artificial intelligence. For instance, using a program to detect AI usage may require students’ work to be processed through an outside third party, which may be a violation of FERPA. In 2023, UC Santa Cruz issued guidance and warned that using services that purport to detect when AI is used in assignments should not be used without disclosure and consent required under FERPA unless certain preconditions were undertaken pertaining to the service having been purchased and vetted by the institution or that the tool is “protected from external access.” "Letter to Faculty about Plagiarism Detection Tools," UC Santa Cruz, 2023, https://ucsc-expghost.imodules.com/controls/email_marketing/view_in_browser.aspx?sid=1069&gid=1001&sendId=4255642&ecatid=4&puid=. Go to reference
The Children's Online Privacy Protection Act (COPPA) sets specific requirements for operators of websites or online services that knowingly collect personal data from children under 13. "Children's Online Privacy Protection Rule ("COPPA")," Federal Trade Commission, 2013, https://www.ftc.gov/legal-library/browse/rules/childrens-online-privacy-protection-rule-coppa. Go to reference Primarily, it requires direct parental notification and parental consent for the collection of these children's personal information and allows parents to control what happens to this data. It establishes that companies that collect this information must have clear policies for what information is collected and how it is secured. Though this would not apply to most high school or postsecondary students, COPPA requirements would apply to many companies that make products for educational use. As such, developers who ignore COPPA guidelines may put themselves in precarious legal and ethical positions.
Though this law was enacted in 1998, there have only been a few changes in the last decade. However, in 2023, the Federal Trade Commission issued a Notice of Proposed Rulemaking for updates to COPPA. Lesley Fair, "FTC Proposes Enhanced Protections for Kids Online. Where Do You Stand?," Federal Trade Commission, 2023, https://www.ftc.gov/business-guidance/blog/2023/12/ftc-proposes-enhanced-protections-kids-online-where-do-you-stand. Go to reference These changes were meant to update COPPA to better reflect evolutions in technology and data practices:
- It includes codifying guidance that schools and school districts can designate developers to use the personal information of students but only when it reflects “school-authorized educational purpose,” not for commercial purposes;
- It would mandate an opt-in to data disclosure when third parties were involved; and
- It would limit the ability to carry out push notifications to encourage more use of the product.
As of April 2024, final updated regulations have not been released, but many of these provisions will likely be included in final regulatory updates.
4. Background Research and Information
It should be understood that AI data models and programming are biased and incomplete, quickly become outdated, and can result in unreliable and harmful results. While biases are nothing new, the scale, power, and speed of AI is. This technology, if not well designed and regulated, holds the potential for White supremacy culture and discriminatory ideas and practices that can proliferate and deepen with new generations of learners.
To mitigate against this scenario and other ethical challenges, artificial intelligence usage requires human oversight, checks, and balances. AI tools must be inclusively developed with all learners in mind, particularly the most marginalized learners. And these tools must be vetted, deployed, and monitored carefully.
Understanding the technology is critical, but it is absolutely essential for all educators and administrators to have ongoing opportunities for the types of professional development described in NEA’s Policy Statement on Safe, Just, and Equitable Schools. That is, educators and administrators must have quality professional opportunities that allow them to develop “cultural competence and responsiveness, including awareness of one’s own implicit biases and trauma, understanding culturally competent pedagogy, and becoming culturally responsive in one’s approach to education and discipline/behavior.” These skills and this knowledge will position educators and administrators to be able to select inclusive AI tools while also applying their pedagogical expertise to ensure the tools are effective and meet the needs of their diverse learners. Further, this knowledge can help educators see and understand biases that may result from AI tools and develop appropriate remedies or approaches to help students succeed.
States, districts, school boards, and higher education institutions should evaluate (and strengthen where necessary) their existing data governance plans prior to adopting AI tools. In addition, schools and higher education institutions must establish transparency protocols and processes that ensure educators, parents, and students are made aware of and understand what AI-enhanced tools are to be used in schools and on campuses and how those tools and their data will be used and protected. This is particularly true for AI tools that monitor or collect sensitive data, such as surveillance or biometric data.
Institutional structures, such as review boards or scheduled audits, should also be put in place to enforce high-quality standards for the use of AI. These structures should include, as interest holders, a diverse set of students, educators, and caregivers. Data collected through AI should be subject to protocols providing transparency about the types of data being collected and how the data is stored, shared, utilized, and protected. These protocols must also clearly articulate whether and to what degree AI is used for any form of monitoring or surveillance in educational settings and how this data will be governed. Additionally, these protocols must ensure the proprietary rights of students and educators in their original work.
As discussed in Section V.A.3, AI-enabled tools that are intended to play any part in assessing/evaluating students or educators or that would have monitoring or surveillance functions should be approached with caution and must be evaluated, understood, and agreed to by appropriate interest holders (including students, educators, and families).
In this section, we outline multiple potential issues with the use of AI in education, including bias, inaccurate or nonsensical outputs, and breaches of data privacy. While these concerns should not halt the adoption of AI, they make it clear that moving ahead with AI should be done with caution and with a plan to evaluate and address tools for potential ethical violations. Guides such as The Ethical Framework for AI in Education, Institute for Ethical AI in Education, The Ethical Framework for AI in Education (2021), https://www.buckingham.ac.uk/wp-content/uploads/2021/03/The-Institute-for-Ethical-AI-in-Education-The-Ethical-Framework-for-AI-in-Education.pdf. Go to reference the EDSAFE AI Alliance’s “SAFE Framework,” "SAFE Benchmarks Framework," EDSAFE AI Alliance, https://www.edsafeai.org/safe. Go to reference and TeachAI’s “Foundational Policy Ideas for AI in Education” "Foundational Policy Ideas for AI in Education," TeachAI, 2024, https://www.teachai.org/policy. Go to reference provide starting points for schools, districts, and higher education institutions, in partnership with educators and their unions, to develop and carry out such plans. Educators and associations, such as the NEA, must be active participants in shaping how legislation and regulations are crafted at the federal, state, and local levels.
a. Data
Data is a broad concept where AI is concerned. Test scores, grades, names of students, and birthdates are commonly used types of data in an educational setting. Another type of data to be aware of in the context of artificial intelligence is biometric data. This type of data is described by the Department of Homeland Security as “a measurable biological (anatomical and physiological) and behavioral characteristic that can be used for automated recognition.” "Biometrics," U.S. Department of Homeland Security, https://www.dhs.gov/biometrics. Go to reference Biometric data is considered sensitive personal information, and it is used with features such as facial recognition, gait analysis, eye tracking, and analyzing hand motion. Examples of AI tools in an educational setting that might utilize biometric data include test monitoring tools and surveillance cameras.
A third type of data to pay attention to is associations generated by computers based on how humans naturally perceive information. In this case, the relationships between data are just as crucial a component of what is collected. For instance, even if a student’s test score data is scrubbed of the individual student demographic details, a geographic-based IP address might still be collected. Using this data, AI could make associations and assumptions about the relationship between the student’s geographic location and their test scores.
The European Union’s comprehensive data privacy regulation, the General Data Protection Regulation (GDPR), denotes that “personal data” includes “information relating to an identified or identifiable natural person (‘data subject’); an identifiable natural person is one who can be identified, directly or indirectly...” "Art. 4 GDPR—Definitions," European Union, https://gdpr.eu/article-4-definitions/. Go to reference The key here is the notion of indirectly. If anonymized data can still contain information that can then be used along with other data to identify individuals or the characteristics of groups of users, then the data is not truly anonymous. Yves-Alexandre de Montjoye et al., "Unique in the Shopping Mall: On the Reidentifiability of Credit Card Metadata," Science 347, no. 6221 (2015), https://doi.org/10.1126/science.1256297. Go to reference
b. Algorithmic Bias and Inaccurate or Nonsensical Outputs
Given that artificial intelligence systems are built by humans and rely on data that are either collected by humans or generated by human-built systems, they are susceptible to the same problems with bias and inaccuracies as humans. Indeed, since AI tools are not human and cannot reason in the same ways that humans do, they are more prone in some cases to these issues.
Furthermore, technology developers are overwhelmingly younger, White, cisgender, heterosexual, male, and people without disabilities. Stack Overflow, 2022 Developer Survey (2022), https://survey.stackoverflow.co/2022/. Go to reference This means that not only will AI technology tend to reflect the perspectives—and biases—of this population, but also that developers themselves may be blind to these concerns. For example, recent research shows that chatbots, such as GPT-4, provide less advantageous outcomes to individuals with names typically associated with racial minorities or women on topics as diverse as car purchases and election outcome predictions. Amit Haim, Alejandro Salinas, and Julian Nyarko, "What's in a Name? Auditing Large Language Models for Race and Gender Bias," arXiv: 2402.14875 (2024), https://doi.org/10.48550/arXiv.2402.14875. Go to reference Models have also demonstrated notable bias against people with disabilities. Pranav Narayanan Venkit, Mukund Srinath, and Shomir Wilson, "Automated Ableism: An Exploration of Explicit Disability Biases in Sentiment and Toxicity Analysis Models," arXiv: 2307.09209 (2023), https://doi.org/10.48550/arXiv.2307.09209. Go to reference
One particular concern for algorithmic bias concerns facial recognition technology, problems with which have even resulted in people being arrested for crimes they did not commit. Khari Johnson, "How Wrongful Arrests Based on AI Derailed 3 Men's Lives," Wired, March 7, 2022, https://www.wired.com/story/wrongful-arrests-ai-derailed-3-mens-lives/. Go to reference Within education, facial recognition technology can be inaccurate and lead to students being identified or disciplined for offenses they were not involved in, and in some cases, it can mean that students aren’t identified or recognized at all. These problems are exacerbated by the overreliance on intense surveillance measures in schools that primarily serve students of color. Jason P. Nance, "Student Surveillance, Racial Inequalities, and Implicit Racial Bias," Emory Law Journal 66, no. 4 (2017), https://scholarlycommons.law.emory.edu/cgi/viewcontent.cgi?article=1093&context=elj. Go to reference Black women, in particular, have the lowest accuracy rate of facial recognition technology, with errors and misidentification in more than 30 percent of cases. Larry Hardesty, "Study Finds Gender and Skin-Type Bias in Commercial Artificial-Intelligence Systems," MIT News (Feb. 11, 2018). https://news.mit.edu/2018/study-finds-gender-skin-type-bias-artificial-intelligence-systems-0212. Go to reference Notably, in September 2023, New York State banned the use of facial recognition technology in schools after determining that the concerns and risks far outweighed the benefits. "NYS Technology Law," New York State, https://its.ny.gov/nys-technology-law. Go to reference
AI utilizing facial, image, and voice recognition also poses significant problems for the disability community, emphasizing the critical need to control disability bias in AI software. Dialects and speech-language differences are often unaccounted for in AI software, Joseph Wilson, "Why AI Will Never Fully Capture Human Language," Sapiens, October 22, 2022, https://www.sapiens.org/language/ai-oral-languages/. Go to reference rendering voice recognition inaccessible to those with speech, language, and voice disorders, such as aphasia. Additionally, facial and image recognition can be discriminatory and inaccessible to individuals with diagnoses such as cleft palate, blindness, and Down syndrome. Disability identities intersect with all other identities, including other marginalized identities such as Indigenous, Black, and LGBTQ+. Therefore, initiatives focusing on applications of AI for individuals with disabilities must acknowledge and address that people who face multiple forms of marginalization encounter increased degrees of AI bias.
Generative AI can also provide output that is simply wrong, which is particularly dangerous given its ability to generate language that sounds entirely plausible to a human audience. Chatbots have been shown to cite articles that don’t exist, provide harmful medical advice, generate historically inaccurate images, and more. For additional examples, see Gary Marcus, "AI Platforms like ChatGPT Are Easy to Use but Also Potentially Dangerous," Scientific American, December 19, 2022, https://www.scientificamerican.com/article/ai-platforms-like-chatgpt-are-easy-to-use-but-also-potentially-dangerous/; Karen Weise and Cade Metz, "When A.I. Chatbots Hallucinate," New York Times, May 1, 2023, https://www.nytimes.com/2023/05/01/business/ai-chatbots-hallucination.html. Go to reference Furthermore, generative AI tools rely on existing and accessible data to produce content. Because of this, AI tools are not always using current data or research Linda Pophal, "Generative AI and Copyright Issues: What You Need to Know," Information Today (2023). Linda Pophal, "Generative AI and Copyright Issues: What You Need to Know," Information Today (2023). https://www.infotoday.com/IT/jul23/Pophal--Generative-AI-and-Copyright-Issues-What-You-Need-to-Know.shtml. Go to reference and may not have access to academic journals behind paywalls, limiting the types of resources they can draw upon. Considering these limitations, educators and students should be cautious of the integrity of AI-generated content. Moreover, the lack of transparency in how and from what sources AI generates content creates difficulties in the ability to reproduce and verify research results. Joseph Crawford et al., "Artificial Intelligence and Authorship Policy: ChatGPT, Bard Bing AI, and beyond," Journal of University Teaching and Learning Practice 20, no. 5 (2023), https://ro.uow.edu.au/cgi/viewcontent.cgi?article=3300&context=jutlp. Go to reference
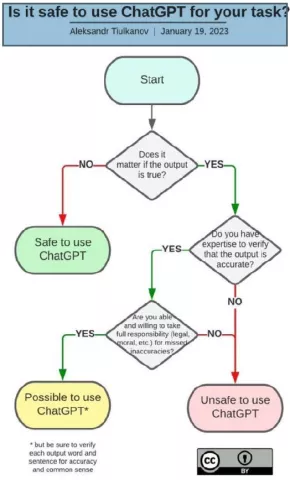
In one widely circulated graphic, an AI and data policy lawyer provides a flowchart of when it is safe to use ChatGPT for a task. The first question is, “Does it matter if the output is true?” If the answer is “Yes,” then one should use Chat GPT—with caution—only if you have the expertise to verify whether the information is accurate and be willing to take responsibility for missed inaccuracies. Clearly, these conditions are very difficult, if not impossible, for younger learners to meet, and even college students may not have the critical thinking and reasoning skills to successfully evaluate generative AI output for accuracy. Aleksandr Tiulkanov, "Is it Safe to Use ChatGPT for Your Task?," 2023, https://www.linkedin.com/posts/tyulkanov_a-simple-algorithm-to-decide-whether-to-use-activity-7021766139605078016-x8Q9. Go to reference
To mitigate the negative effects of algorithmic bias and inaccurate or nonsensical output on educators and students, developers must implement measures to assess and prevent discriminatory or inaccurate outputs, including recruiting a diverse pool of developers and leaders. Developers should also institute diverse and intersectional review boards for the comprehensive evaluation of AI software. This approach not only enhances the overall fairness of the evaluation process but also helps in identifying and rectifying biases that may disproportionately affect people of color, women, LGBTQ+ individuals, and individuals with disabilities.
Actively involving people with disabilities in the development, design, and maintenance of AI systems ensures technology that is not only compliant with accessibility standards but also genuinely user-centric, considering the unique challenges and needs of individuals with disabilities. Furthermore, genuine co-design is essential, incorporating individuals with disabilities within the design team and throughout the design process. This collaborative effort should involve a diverse representation of people with various disabilities. Peter Smith and Laura Smith, "Artificial Intelligence and Disability: Too Much Promise, Yet Too Little Substance?," AI and Ethics 1 (2020), https://doi.org/10.1007/s43681-020-00004-5. Go to reference Involving people with disabilities in the maintenance of artificial intelligence is not just a matter of compliance or ethical consideration; it is essential for creating technology that is truly inclusive, user-friendly, and beneficial for a diverse range of individuals.
Public procurement standards should also be established that are compliant with human rights principles and inclusive of people with disabilities. When procuring AI software, public education agencies must be sure to assess the software against Web Content Accessibility Guidelines (WCAG) "WCAG 2 Overview," W3C Web Accessibility Initiative (WAI), 2024, https://www.w3.org/WAI/standards-guidelines/wcag/. Go to reference and Universal Design for Learning (UDL) Guidelines "UDL: The UDL Guidelines." Go to reference to ensure accessibility to students and educators with disabilities and appropriateness in terms of creating flexible and inclusive learning environments. Public education institutions must take a proactive stance against discrimination, embedding human rights principles into regulations governing AI development and deployment.
Additionally, AI tools should be monitored and assessed regularly, and educators should be trained to help identify, report, and address AI bias and inaccuracies and provide the knowledge and skills to educate their students on how to identify biases and inaccuracies. Any and all AI systems that schools, districts, or states are considering using in classrooms or school buildings should be vetted, tested, and monitored for all potential biases and inaccuracies, and strict protocols should be implemented with input from all education interest holders to ensure these tools are ethically designed and implemented to keep schools safe without harming students and educators.
c. Ethical Issues with AI Usage
Beyond issues with bias and inaccuracies, AI presents a number of ethical dilemmas concerning its use in surveillance, its threats to academic integrity and intellectual property rights, and its ability to provide new avenues for bullying and harassment.
Surveillance
Artificial intelligence can parse large amounts of data and identify patterns much more quickly than current technology. For some schools, districts, or institutions, this AI may be utilized as a way to monitor both students and staff—for safety, policy enforcement, assessments, or content moderation. While these technological uses may have benefits, care must be taken to ensure the accuracy and validity of the data, consider additional contextual and unique information about the individual that should be taken into account, and ensure that the technology and its resulting data are used in a manner that supports a human-centered approach to education.
While the NEA recognizes that cameras (including CCTV cameras) are commonly used by many institutions, including schools and higher education institutions, for security, we are concerned that AI-enabled surveillance, such as gait recognition and iris scans, could result in erroneous data that could be used for highly consequential decisions. Furthermore, tools that purport to track in-classroom engagement or focus by analyzing eye movement and facial expressions may have the unintended consequence of students becoming more aware of their own facial expressions and focus and may lead to self-censoring their expressions. Mark Andrejevic and Neil Selwyn, "Facial Recognition Technology in Schools: Critical Questions and Concerns," Learning, Media and Technology 45, no. 2 (2020), https://www.tandfonline.com/doi/full/10.1080/17439884.2020.1686014. Go to reference This may lead to students being unwilling to engage authentically and instead using more performative responses that they know will meet expectations from these programs.
Surveillance technologies, such as remote proctoring systems, can be especially discriminatory toward those with disabilities. The Center for Democracy & Technology published a guide in May 2022 on ableism and disability discrimination in education-related surveillance technologies and noted that individuals with disabilities are more likely to be flagged as potentially suspicious by this software due to their disability-specific access needs, such as needing longer breaks or using screen readers or dictation software. Lydia X. Z. Brown et al., Ableism And Disability Discrimination in New Surveillance Technologies, Center for Democracy & Technology (2022), https://cdt.org/wp-content/uploads/2022/05/2022-05-23-CDT-Ableism-and-Disability-Discrimination-in-New-Surveillance-Technologies-report-final-redu.pdf. Go to reference
Using AI to track educator web access also represents a threat to academic freedom and could create a chilling effect on the online speech and expression of students and educators. For many disciplines, conducting academic research may require access to sites or resources that may go against institutional network terms of service. Some institutions may have processes for allowing faculty or researchers access when needed, but the process may be done through manual approval, which may not be possible with AI tools like generative AI chatbots.
Intellectual Property Rights
The use of generative AI poses various challenges for students and educators in both pre-K–12 and higher education regarding proprietary rights, intellectual property (IP), and copyright infringement within teaching, learning, and research. Beck Wise et al., "A Scholarly Dialogue: Writing Scholarship, Authorship, Academic Integrity and the Challenges of AI," Higher Education Research & Development 43, no. 3 (2024), https://doi.org/10.1080/07294360.2023.2280195. Go to reference
- Copyrighting Generated Content: Students and educators at all levels are actively generating and using content for teaching and learning without clear guidance or knowledge of potential legal ramifications. A primary challenge is determining ownership of AI-generated content. Copyright laws are based on human authorship, raising concerns about who has the right to claim ownership and how creators can protect their works that are generated by AI tools. U.S. Copyright Office, Library of Congress, Copyright Registration Guidance: Works Containing Material Generated by Artificial Intelligence (2023), https://www.federalregister.gov/documents/2023/03/16/2023-05321/copyright-registration-guidance-works-containing-material-generated-by-artificial-intelligence. Go to reference The United States Copyright Office defines proprietary rights in terms of human creativity, excluding non-humans. U.S. Copyright Office, Copyright Registration Guidance: Works Containing Material Generated by Artificial Intelligence. Go to reference This presents a legal and philosophical quandary on whether AI-generated materials can or should be protected under current copyright laws. Mala Chatterjee and Jeanne C. Fromer, "Minds, Machines, and the Law: The Case of Volition in Copyright Law," Columbia Law Review 119, no. 7 (2019), https://columbialawreview.org/content/minds-machines-and-the-law-the-case-of-volition-in-copyright-law/. Go to reference Arguments have emerged stating that if AI is viewed as a tool, like other computer software, then AI-generated materials should be allowed protection. However, if AI tools are used to generate materials subject to copyright, then AI companies may have an ownership claim. Christopher T. Zirpoli, Generative Artificial Intelligence and Copyright Law, Congressional Research Service (2023), https://crsreports.congress.gov/product/pdf/LSB/LSB10922. Go to reference
- Copyright and Intellectual Property Infringement: Generative AI tools are typically trained using existing, human-created knowledge and artifacts to produce content. Pophal, "Generative AI and Copyright Issues: What You Need to Know."; Zirpoli, Generative Artificial Intelligence and Copyright Law. Go to reference Therefore, AI tools can generate content that is based on or resembles copyrighted materials. This raises concerns about copyright infringement, especially when AI-generated content is used without proper licensing or permission. If AI-generated materials infringe on existing materials, then the question arises of who is at fault—the individual who prompted the AI tool to generate the content or the company that created the AI tool that was potentially trained on copyrighted material. Chatterjee and Fromer, "Minds, Machines, and the Law: The Case of Volition in Copyright Law." Go to reference Regardless, educators and students generating content with AI tools must be aware that they may be held accountable for violating copyright and IP laws. Existing intellectual property laws may be inadequate to address the challenges posed by generative AI technologies. Policymakers, collaborating with academia and legal experts, must update laws to protect the rights of creators and ensure fair use of AI-generated content.
It is crucial that higher educational institutions and school districts, in partnership with associations, educators, faculty, and students, adopt and implement policies that clearly define the acceptable use of AI tools and materials for teaching and learning across all subject areas that will protect proprietary rights, respect intellectual property, and deter copyright infringement. Additionally, educational institutions and academic associations, in partnership with higher education faculty, must develop and implement guidance for acceptable and ethical research practices using AI.
Academic Integrity
A notable concern among educators at all levels is the temptation for many students to use AI tools to plagiarize or cheat on written assignments. The ease of access to generative AI tools may be viewed as an institutional-wide threat to academic integrity. Tomas Foltynek et al., "ENAI Recommendations on the Ethical use of Artificial Intelligence in Education," International Journal for Educational Integrity 19, no. 1 (2023), https://doi.org/10.1007/s40979-023-00133-4. Go to reference Due to the sudden emergence of generative AI tools in teaching and learning, educators and students at all levels find themselves struggling to define and identify academic misconduct.
Notably, the use of AI detection software poses a second challenge to academic misconduct. First, biased AI cheating detection applications have incorrectly flagged students for misconduct. For instance, emergent multilingual learners have been falsely accused of submitting written assignments using AI-generated content because AI detection software is largely trained using writing samples from native English speakers. Weixin Liang et al., "GPT detectors are biased against non-native English writers," Patterns 4, no. 7 (2023), https://doi.org/10.1016/j.patter.2023.100779. Go to reference Additionally, facial recognition technology used in AI cheating detection software is biased toward White cisgender males, decreasing the accuracy in detecting misconduct among students of color, cisgender females, transgender individuals, and students with disabilities. Brown et al., Ableism And Disability Discrimination in New Surveillance Technologies; Steven Feldstein, "Types of AI Surveillance," in The Global Expansion of AI Surveillance (Carnegie Endowment for International Peace, 2019); Holmes, The Unintended Consequences of Artificial Intelligence and Education; Kashyap Kompella, "Transgender Bias in AI," Information Today 39, no. 4 (2022), https://research.ebsco.com/linkprocessor/plink?id=e68e5ca7-b8de-3dda-8f74-25a101669832; Jo Ann Oravec, "AI, Biometric Analysis, and Emerging Cheating Detection Systems: The Engineering of Academic Integrity?," Education Policy Analysis Archives 30 (2022), https://epaa.asu.edu/index.php/epaa/article/view/5765. Go to reference Moreover, studies have shown that AI detection tools are largely inaccurate and unreliable in differentiating between AI-generated and human-written content. Ahmed M. Elkhatat, Khaled Elsaid, and Saeed Almeer, "Evaluating the Efficacy of AI Content Detection Tools in Differentiating between Human and AI-Generated Text," International Journal for Educational Integrity 19, no. 1 (2023), https://doi.org/10.1007/s40979-023-00140-5; Debora Weber-Wulff et al., "Testing of Detection Tools for AI-Generated Text," International Journal for Educational Integrity 19, no. 1 (2023), https://doi.org/10.1007/s40979-023-00146-z. Go to reference
The Task Force believes that educational institutions, in partnership with educators and students, must create clear learning objectives that identify how AI may be used for assignments and how using AI could impact learning objectives. Clear guidelines can help educators and students navigate the acceptable use of AI tools to support teaching and learning while mitigating threats of misconduct. Ella T. August, Olivia S. Anderson, and Frederique A. Laubepin, "Brave New Words: A Framework and Process for Developing Technology-Use Guidelines for Student Writing," Pedagogy in Health Promotion (2024), https://journals.sagepub.com/doi/full/10.1177/23733799241235119. Go to reference
Bullying and Harassment
When it comes to bullying and harassment among students, AI has been offered as both a preventive measure and a facilitator of greater harm. Artificial intelligence algorithms, when built into apps and other systems, can be used to quickly identify and shut down abusive messages and even provide victims with customized support. Sameer Hinduja, "How Machine Learning Can Help Us Combat Online Abuse: A Primer," Cyberbullying Research Center. https://cyberbullying.org/machine-learning-can-help-us-combat-online-abuse-primer; Elena Sidorova, "Stop Cyberbullying with Artificial Intelligence," KidActions, 2022, https://www.kidactions.eu/2022/08/04/artificial-intelligence/. Go to reference Yet, these systems are not infallible—cyberbullying and harassment may use emojis in place of text or harmful words or new slang that are yet to be considered problematic; therefore, they may not be part of the detection system. Hinduja, "How Machine Learning Can Help Us Combat Online Abuse: A Primer." Go to reference Furthermore, AI systems do not understand the context in which language is used, particularly subtleties such as sarcasm and wit, which may lead to incorrectly tagging non-harmful content as problematic or missing content that is actually abusive. Hinduja, "How Machine Learning Can Help Us Combat Online Abuse: A Primer." Go to reference
At the same time, AI has emerged as a new tool to facilitate bullying and harassment. Reports have proliferated in the United States Natasha Singer, "Teen Girls Confront an Epidemic of Deepfake Nudes in Schools," New York Times, April 8, 2024, https://www.nytimes.com/2024/04/08/technology/deepfake-ai-nudes-westfield-high-school.html. Go to reference and abroad "AI Becomes the Newest Weapon in the School Bully Arsenal," OECD.AI Policy Observatory AI Incidents Monitor, accessed April 21, 2024, https://oecd.ai/en/incidents/38444. Go to reference of students using generative AI to create sexually explicit and pornographic ‘deepfake’ images of peers. While these are the highest-profile incidents, AI can also be used to bombard victims with personalized harassing messages, convince people that they are interacting with someone they are not (i.e., ‘catfishing’), or proliferate hate speech. Sameer Hinduja, "Generative AI as a Vector for Harassment and Harm," Cyberbullying Research Center (2023). https://cyberbullying.org/generative-ai-as-a-vector-for-harassment-and-harm. Go to reference
Many education institutions were caught unprepared to handle these incidents. Singer, "Teen Girls Confront an Epidemic of Deepfake Nudes in Schools." Go to reference The Federal Bureau of Investigation recently clarified that using generative AI to create child sexual abuse material is illegal, "Child Sexual Abuse Material Created by Generative AI and Similar Online Tools is Illegal," Federal Bureau of Investigation, 2024, https://www.ic3.gov/Media/Y2024/PSA240329. Go to reference and legislation in this area is moving through Congress and some state legislatures. Alyson Klein, "What a Proposed Ban on AI-Assisted ‘Deep Fakes’ Would Mean for Cyberbullying," Education Week, January 12, 2024, https://www.edweek.org/policy-politics/what-a-proposed-ban-on-ai-assisted-deep-fakes-would-mean-for-cyberbullying/2024/01. Go to reference Yet, some advocacy groups have cautioned against placing too many limitations on AI-generated content, lest there be infringements on free expression and fair use. American Civil Liberties Union et al., "Letter to Representative Darrell Issa and Representative Hank Johnson," (Feb. 1, 2024). https://cdt.org/wp-content/uploads/2024/02/Coalition-Letter-NO-AI-Fraud-Act-_-NO-FAKES-Act-2.1.2024-.pdf; Katherine Klosek, "No Frauds, No Fakes…No Fair Use?," Association of Research Librarians, March 1, 2024, https://www.arl.org/blog/nofraudsnofakes/. Go to reference While these larger debates are being settled, the Task Force believes that schools and higher education institutions should protect students and educators by updating their codes of conduct and other bullying and harassment policies to encompass the use of AI in these contexts.
d. Data Privacy and Security
AI tools should be designed to collect the minimum amount of personal data needed, and, to the extent possible or required by law, data and metadata should be limited to what is necessary to accomplish the task. In the context of education, we must consider users as students, educators, administrators, and families. Similar to the provisions in the European GDPR, we believe that AI tools should process only the required minimum necessary data for each specific purpose and have mechanisms for being able to decline other types of data collection. In the case of educational software, we must consider that the data collected may have unique legal and moral considerations. Breaking down the types of collected data between necessary and optional is crucial for evaluating data use policies:
- Required Data: Some data and uses may be required for data functionality—for instance, collecting IP address and device ID information or unique identifiers, such as a birthdate or name.
- Optional Data: This data could include data collected for analytical purposes, bonus features, or cross-platform tracking identifiers not required for primary functionality. Opting out of some functionality or data collection may limit one's ability to use the full capabilities of the software.
Given that AI cannot operate without data—and often very large amounts of highly sensitive data—the growing prevalence of these tools further exposes education institutions to data privacy and security threats. Education institutions are particularly attractive to cybercriminals because they hold unique datasets that include both students and their families, including highly sensitive data, such as student health data, Social Security numbers, and families’ credit card data. Frederick Hess, "The Top Target For Ransomware? It’s Now K–12 Schools," Forbes, Sept. 23, 2023, https://www.forbes.com/sites/frederickhess/2023/09/20/the-top-target-for-ransomware-its-now-k-12-schools/. Go to reference Higher education institutions are also more likely than entities in other sectors to pay a ransom. Sophos, The State of Ransomware in Education 2023 (2023), https://assets.sophos.com/X24WTUEQ/at/j74v496cfwh4qsvgqhs4pmw/sophos-state-of-ransomware-education-2023-wp.pdf. Go to reference The U.S. Government Accountability Office further noted that while the U.S. Department of Education provides cybersecurity preparedness resources, For example: "Cybersecurity Preparedness for Schools and Institutions of Higher Education," U.S. Department of Education, Readiness and Emergency Management for Schools Technical Assistance Center, 2024, https://rems.ed.gov/Cyber. Go to reference there is little coordination among agencies or with the education community about this issue, nor are there any measures of the effectiveness of the cybersecurity products and services the federal government provides. U.S. Government Accountability Office, Critical Infrastructure Protection: Additional Federal Coordination Is Needed to Enhance K–12 Cybersecurity (2022), https://www.gao.gov/products/gao-23-105480. Go to reference
It is not surprising, then, that the education sector has become a target for cybercriminals. One cybersecurity firm estimates that the minimum number of U.S. pre-K–12 districts that were impacted by ransomware more than doubled from 45 in 2022 to 108 in 2023. Emsisoft, The State of Ransomware in the U.S.: Report and Statistics 2023 (2024), https://www.emsisoft.com/en/blog/44987/the-state-of-ransomware-in-the-u-s-report-and-statistics-2023/. Go to reference Among the 108 districts, 77 had data stolen, affecting 1,899 schools. Threats against higher education institutions also jumped, from 44 in 2022 to 72 in 2023, with 60 having data stolen. Combining the pre-K–12 and higher education data, the education sector outpaces both health care and government in terms of data security threats. A similar survey conducted worldwide found that an astounding 80 percent of pre-K–12 providers and 79 percent of higher education institutions experienced ransomware attacks, costing millions of dollars in recovery costs. Sophos, The State of Ransomware in Education 2023. Go to reference
Transparency is instrumental in protecting students and educators from data harms. To ensure transparency, educators at all levels must be involved in the decision-making process regarding AI vetting, adoption, and deployment. Additionally, the Task Force calls on school districts and postsecondary institutions to inform students, educators, and families about which AI technologies are implemented, the intended benefits of those tools, the data they require, and the protocols in place to collect, store, and utilize those data. In states with collective bargaining rights, educator contracts should include provisions for data privacy and security.
Some organizations, such as EDSAFE AI Alliance, have already created guidance "Consultancy Protocol for Building AI Capacity in Your School District," EDSAFE AI Alliance, https://drive.google.com/file/d/1-u7uq0dvSB7IddXR2hVv-KTezpCK_ic_/view. Go to reference on district consultancy protocols for AI implementation, which include:
- Analysis of the current state of AI readiness within the district;
- Assessment and action planning, including needs assessment and analysis of equity, safety, and ethical considerations;
- Action planning, including professional learning, communication and engagement, and governance and oversight; and
- Additional considerations that focus on data privacy, security, transparency, and accountability.
Recognizing that every district may have different resources, composition, and needs, this represents more of a framework and is designed to be adopted based on specific details of each individual district or higher education institution.
e. The Environmental Impact of Artificial Intelligence
One of the major takeaways from the U.S. Global Change Research Program’s Fifth National Climate Assessment from fall 2023 is that the United States is warming faster than the rest of the world due to human activity. U.S. Global Change Research Program, Fifth National Climate Assessment (2024), https://nca2023.globalchange.gov/. Go to reference Negative impacts of climate change have undue and unequal consequences on Native, Asian, Black, Latin(o/a/x), Middle Eastern and North African, Multiracial, Pacific Islander, and other communities of color, under-resourced urban and rural communities, people with disabilities, and girls and women. While the connection is not inherent, it is important that decision-makers and policymakers acknowledge, consider, and confront the environmental impacts of artificial intelligence and cloud technology. Joseph B. Keller, Manann Donoghoe, and Andre M. Perry, The US Must Balance Climate Justice Challenges in the Era of Artificial Intelligence, Brookings Institution (2024), https://www.brookings.edu/articles/the-us-must-balance-climate-justice-challenges-in-the-era-of-artificial-intelligence/. Go to reference “In the race to produce faster and more accurate AI models, environmental sustainability is often regarded as a second-class citizen,” noted University of Florence Assistant Professor Roberto Verdecchia. Keller, Donoghoe, and Perry, The US Must Balance Climate Justice Challenges in the Era of Artificial Intelligence. Go to reference
Although these technologies operate in virtual spaces, AI and the cloud will intensify greenhouse gas emissions, consume increasing amounts of energy, and require larger quantities of natural resources. Keller, Donoghoe, and Perry, The US Must Balance Climate Justice Challenges in the Era of Artificial Intelligence. Go to reference Research suggests that a single generative AI query consumes energy at four or five times the magnitude of a typical search engine request, and image-generating tasks are even more energy-intensive. Since 2012, the most extensive AI training runs have used exponentially more computing power, doubling every 3.4 months, on average. Niklas Sundberg, "Tackling AI’s Climate Change Problem," MIT Sloan Management Review, 2024, https://sloanreview.mit.edu/article/tackling-ais-climate-change-problem/. Go to reference For example, generating a single image using a powerful AI model consumes as much energy as fully charging your smartphone. Melissa Heikkilä, "Making an Image with Generative AI Uses as Much Energy as Charging Your Phone," Technology Review (Dec. 1, 2023). https://www.technologyreview.com/2023/12/01/1084189/making-an-image-with-generative-ai-uses-as-much-energy-as-charging-your-phone/. Go to reference Even more alarming is that training a single large AI model can emit more than 626,000 pounds of carbon dioxide, which is nearly five times the lifetime emissions of the average American car (inclusive of the manufacturing of the car itself). Karen Hao, "Training a Single AI Model Can Emit as Much Carbon as Five Cars in their Lifetimes," MIT Technology Review (June 6, 2019). https://www.technologyreview.com/2019/06/06/239031/training-a-single-ai-model-can-emit-as-much-carbon-as-five-cars-in-their-lifetimes/. Go to reference
With the increasing need for computing power, new data centers are being built across the country. Many of these centers are built in rural areas that have lower land valuations compared to suburban or urban areas. Additionally, these data centers need to compete not only for energy but also for local natural resources, like water. The immense processing power of these data centers generates an enormous amount of heat as a byproduct, which requires methods for substantial cooling. The most common method requires a large amount of water and electricity to cool the data center.
Up to one-fifth of data center servers draw water directly from “moderately to highly water-stressed areas.” Md Abu Bakar Siddik, Arman Shehabi, and Landon Marston, "The Environmental Footprint of Data Centers in the United States," Environmental Research Letters 16 (2021), https://doi.org/10.1088/1748-9326/abfba1. Go to reference Power sources with low carbon footprints, like solar or wind power, are predominantly in areas that have lower water resources. Areas like Utah, Arizona, and Nevada, which have seen enormous growth in data centers, are also some of the highest water-stressed areas. We also see multiple equity issues emerge as pollution from power generation facilities can impact local air and water quality. Data centers may also contribute to increased electricity costs, as demand in local markets may make electricity more expensive for all, with the impact felt especially for those with the lowest income and wealth.
While it is nearly impossible for researchers to evaluate the full extent of the negative environmental impacts of AI technologies, decision-makers in educational settings should be mindful of their environmental impacts throughout the planning and implementation phases.
- 53 "California Consumer Privacy Act (CCPA)," State of California, Department of Justice, updated March 13, 2024, https://oag.ca.gov/privacy/ccpa.
- 54 "Code of Virginia—Chapter 53. Consumer Data Protection Act," Virginia Law, 2021, https://law.lis.virginia.gov/vacodefull/title59.1/chapter53/.
- 55 U.S. Department of Health and Human Services, Office of the U.S. Surgeon General, Social Media and Youth Mental Health (2023), https://www.hhs.gov/surgeongeneral/priorities/youth-mental-health/social-media/index.html.
- 56 Committee on Energy and Commerce, "Committee Chairs Rodgers, Cantwell Unveil Historic Draft Comprehensive Data Privacy Legislation," news release, April 7, 2024, https://energycommerce.house.gov/posts/committee-chairs-rodgers-cantwell-unveil-historic-draft-comprehensive-data-privacy-legislation.
- 57 "H.R.8152 – 117th Congress (2021–2022): American Data Privacy and Protection Act," U.S. House of Representatives, 2022, https://www.congress.gov/bill/117th-congress/house-bill/8152.
- 58 The White House, "Executive Order on the Safe, Secure, and Trustworthy Development and Use of Artificial Intelligence."
- 59 "Family Educational Rights and Privacy Act (FERPA)," U.S. Department of Education, 2021, https://www2.ed.gov/policy/gen/guid/fpco/ferpa/index.html.
- 60 "Letter to Faculty about Plagiarism Detection Tools," UC Santa Cruz, 2023, https://ucsc-expghost.imodules.com/controls/email_marketing/view_in_browser.aspx?sid=1069&gid=1001&sendId=4255642&ecatid=4&puid=.
- 61 "Children's Online Privacy Protection Rule ("COPPA")," Federal Trade Commission, 2013, https://www.ftc.gov/legal-library/browse/rules/childrens-online-privacy-protection-rule-coppa.
- 62 Lesley Fair, "FTC Proposes Enhanced Protections for Kids Online. Where Do You Stand?," Federal Trade Commission, 2023, https://www.ftc.gov/business-guidance/blog/2023/12/ftc-proposes-enhanced-protections-kids-online-where-do-you-stand.
- 63 Institute for Ethical AI in Education, The Ethical Framework for AI in Education (2021), https://www.buckingham.ac.uk/wp-content/uploads/2021/03/The-Institute-for-Ethical-AI-in-Education-The-Ethical-Framework-for-AI-in-Education.pdf.
- 64 "SAFE Benchmarks Framework," EDSAFE AI Alliance, https://www.edsafeai.org/safe.
- 65 "Foundational Policy Ideas for AI in Education," TeachAI, 2024, https://www.teachai.org/policy.
- 66 "Biometrics," U.S. Department of Homeland Security, https://www.dhs.gov/biometrics.
- 67 "Art. 4 GDPR—Definitions," European Union, https://gdpr.eu/article-4-definitions/.
- 68 Yves-Alexandre de Montjoye et al., "Unique in the Shopping Mall: On the Reidentifiability of Credit Card Metadata," Science 347, no. 6221 (2015), https://doi.org/10.1126/science.1256297.
- 69 Stack Overflow, 2022 Developer Survey (2022), https://survey.stackoverflow.co/2022/.
- 70 Amit Haim, Alejandro Salinas, and Julian Nyarko, "What's in a Name? Auditing Large Language Models for Race and Gender Bias," arXiv: 2402.14875 (2024), https://doi.org/10.48550/arXiv.2402.14875.
- 71 Pranav Narayanan Venkit, Mukund Srinath, and Shomir Wilson, "Automated Ableism: An Exploration of Explicit Disability Biases in Sentiment and Toxicity Analysis Models," arXiv: 2307.09209 (2023), https://doi.org/10.48550/arXiv.2307.09209.
- 72 Khari Johnson, "How Wrongful Arrests Based on AI Derailed 3 Men's Lives," Wired, March 7, 2022, https://www.wired.com/story/wrongful-arrests-ai-derailed-3-mens-lives/.
- 73 Jason P. Nance, "Student Surveillance, Racial Inequalities, and Implicit Racial Bias," Emory Law Journal 66, no. 4 (2017), https://scholarlycommons.law.emory.edu/cgi/viewcontent.cgi?article=1093&context=elj.
- 74 Larry Hardesty, "Study Finds Gender and Skin-Type Bias in Commercial Artificial-Intelligence Systems," MIT News (Feb. 11, 2018). https://news.mit.edu/2018/study-finds-gender-skin-type-bias-artificial-intelligence-systems-0212.
- 75 "NYS Technology Law," New York State, https://its.ny.gov/nys-technology-law.
- 76 Joseph Wilson, "Why AI Will Never Fully Capture Human Language," Sapiens, October 22, 2022, https://www.sapiens.org/language/ai-oral-languages/.
- 77 For additional examples, see Gary Marcus, "AI Platforms like ChatGPT Are Easy to Use but Also Potentially Dangerous," Scientific American, December 19, 2022, https://www.scientificamerican.com/article/ai-platforms-like-chatgpt-are-easy-to-use-but-also-potentially-dangerous/; Karen Weise and Cade Metz, "When A.I. Chatbots Hallucinate," New York Times, May 1, 2023, https://www.nytimes.com/2023/05/01/business/ai-chatbots-hallucination.html.
- 78 Linda Pophal, "Generative AI and Copyright Issues: What You Need to Know," Information Today (2023). Linda Pophal, "Generative AI and Copyright Issues: What You Need to Know," Information Today (2023). https://www.infotoday.com/IT/jul23/Pophal--Generative-AI-and-Copyright-Issues-What-You-Need-to-Know.shtml.
- 79 Joseph Crawford et al., "Artificial Intelligence and Authorship Policy: ChatGPT, Bard Bing AI, and beyond," Journal of University Teaching and Learning Practice 20, no. 5 (2023), https://ro.uow.edu.au/cgi/viewcontent.cgi?article=3300&context=jutlp.
- 80 Aleksandr Tiulkanov, "Is it Safe to Use ChatGPT for Your Task?," 2023, https://www.linkedin.com/posts/tyulkanov_a-simple-algorithm-to-decide-whether-to-use-activity-7021766139605078016-x8Q9.
- 81 Peter Smith and Laura Smith, "Artificial Intelligence and Disability: Too Much Promise, Yet Too Little Substance?," AI and Ethics 1 (2020), https://doi.org/10.1007/s43681-020-00004-5.
- 82 "WCAG 2 Overview," W3C Web Accessibility Initiative (WAI), 2024, https://www.w3.org/WAI/standards-guidelines/wcag/.
- 83 "UDL: The UDL Guidelines."
- 84 Mark Andrejevic and Neil Selwyn, "Facial Recognition Technology in Schools: Critical Questions and Concerns," Learning, Media and Technology 45, no. 2 (2020), https://www.tandfonline.com/doi/full/10.1080/17439884.2020.1686014.
- 85 Lydia X. Z. Brown et al., Ableism And Disability Discrimination in New Surveillance Technologies, Center for Democracy & Technology (2022), https://cdt.org/wp-content/uploads/2022/05/2022-05-23-CDT-Ableism-and-Disability-Discrimination-in-New-Surveillance-Technologies-report-final-redu.pdf.
- 86 Beck Wise et al., "A Scholarly Dialogue: Writing Scholarship, Authorship, Academic Integrity and the Challenges of AI," Higher Education Research & Development 43, no. 3 (2024), https://doi.org/10.1080/07294360.2023.2280195.
- 87 U.S. Copyright Office, Library of Congress, Copyright Registration Guidance: Works Containing Material Generated by Artificial Intelligence (2023), https://www.federalregister.gov/documents/2023/03/16/2023-05321/copyright-registration-guidance-works-containing-material-generated-by-artificial-intelligence.
- 88 U.S. Copyright Office, Copyright Registration Guidance: Works Containing Material Generated by Artificial Intelligence.
- 89 Mala Chatterjee and Jeanne C. Fromer, "Minds, Machines, and the Law: The Case of Volition in Copyright Law," Columbia Law Review 119, no. 7 (2019), https://columbialawreview.org/content/minds-machines-and-the-law-the-case-of-volition-in-copyright-law/.
- 90 Christopher T. Zirpoli, Generative Artificial Intelligence and Copyright Law, Congressional Research Service (2023), https://crsreports.congress.gov/product/pdf/LSB/LSB10922.
- 91 Pophal, "Generative AI and Copyright Issues: What You Need to Know."; Zirpoli, Generative Artificial Intelligence and Copyright Law.
- 92 Chatterjee and Fromer, "Minds, Machines, and the Law: The Case of Volition in Copyright Law."
- 93 Tomas Foltynek et al., "ENAI Recommendations on the Ethical use of Artificial Intelligence in Education," International Journal for Educational Integrity 19, no. 1 (2023), https://doi.org/10.1007/s40979-023-00133-4.
- 94 Weixin Liang et al., "GPT detectors are biased against non-native English writers," Patterns 4, no. 7 (2023), https://doi.org/10.1016/j.patter.2023.100779.
- 95 Brown et al., Ableism And Disability Discrimination in New Surveillance Technologies; Steven Feldstein, "Types of AI Surveillance," in The Global Expansion of AI Surveillance (Carnegie Endowment for International Peace, 2019); Holmes, The Unintended Consequences of Artificial Intelligence and Education; Kashyap Kompella, "Transgender Bias in AI," Information Today 39, no. 4 (2022), https://research.ebsco.com/linkprocessor/plink?id=e68e5ca7-b8de-3dda-8f74-25a101669832; Jo Ann Oravec, "AI, Biometric Analysis, and Emerging Cheating Detection Systems: The Engineering of Academic Integrity?," Education Policy Analysis Archives 30 (2022), https://epaa.asu.edu/index.php/epaa/article/view/5765.
- 96 Ahmed M. Elkhatat, Khaled Elsaid, and Saeed Almeer, "Evaluating the Efficacy of AI Content Detection Tools in Differentiating between Human and AI-Generated Text," International Journal for Educational Integrity 19, no. 1 (2023), https://doi.org/10.1007/s40979-023-00140-5; Debora Weber-Wulff et al., "Testing of Detection Tools for AI-Generated Text," International Journal for Educational Integrity 19, no. 1 (2023), https://doi.org/10.1007/s40979-023-00146-z.
- 97 Ella T. August, Olivia S. Anderson, and Frederique A. Laubepin, "Brave New Words: A Framework and Process for Developing Technology-Use Guidelines for Student Writing," Pedagogy in Health Promotion (2024), https://journals.sagepub.com/doi/full/10.1177/23733799241235119.
- 98 Sameer Hinduja, "How Machine Learning Can Help Us Combat Online Abuse: A Primer," Cyberbullying Research Center. https://cyberbullying.org/machine-learning-can-help-us-combat-online-abuse-primer; Elena Sidorova, "Stop Cyberbullying with Artificial Intelligence," KidActions, 2022, https://www.kidactions.eu/2022/08/04/artificial-intelligence/.
- 99 Hinduja, "How Machine Learning Can Help Us Combat Online Abuse: A Primer."
- 100 Hinduja, "How Machine Learning Can Help Us Combat Online Abuse: A Primer."
- 101 Natasha Singer, "Teen Girls Confront an Epidemic of Deepfake Nudes in Schools," New York Times, April 8, 2024, https://www.nytimes.com/2024/04/08/technology/deepfake-ai-nudes-westfield-high-school.html.
- 102 "AI Becomes the Newest Weapon in the School Bully Arsenal," OECD.AI Policy Observatory AI Incidents Monitor, accessed April 21, 2024, https://oecd.ai/en/incidents/38444.
- 103 Sameer Hinduja, "Generative AI as a Vector for Harassment and Harm," Cyberbullying Research Center (2023). https://cyberbullying.org/generative-ai-as-a-vector-for-harassment-and-harm.
- 104 Singer, "Teen Girls Confront an Epidemic of Deepfake Nudes in Schools."
- 105 "Child Sexual Abuse Material Created by Generative AI and Similar Online Tools is Illegal," Federal Bureau of Investigation, 2024, https://www.ic3.gov/Media/Y2024/PSA240329.
- 106 Alyson Klein, "What a Proposed Ban on AI-Assisted ‘Deep Fakes’ Would Mean for Cyberbullying," Education Week, January 12, 2024, https://www.edweek.org/policy-politics/what-a-proposed-ban-on-ai-assisted-deep-fakes-would-mean-for-cyberbullying/2024/01.
- 107 American Civil Liberties Union et al., "Letter to Representative Darrell Issa and Representative Hank Johnson," (Feb. 1, 2024). https://cdt.org/wp-content/uploads/2024/02/Coalition-Letter-NO-AI-Fraud-Act-_-NO-FAKES-Act-2.1.2024-.pdf; Katherine Klosek, "No Frauds, No Fakes…No Fair Use?," Association of Research Librarians, March 1, 2024, https://www.arl.org/blog/nofraudsnofakes/.
- 108 Frederick Hess, "The Top Target For Ransomware? It’s Now K–12 Schools," Forbes, Sept. 23, 2023, https://www.forbes.com/sites/frederickhess/2023/09/20/the-top-target-for-ransomware-its-now-k-12-schools/.
- 109 Sophos, The State of Ransomware in Education 2023 (2023), https://assets.sophos.com/X24WTUEQ/at/j74v496cfwh4qsvgqhs4pmw/sophos-state-of-ransomware-education-2023-wp.pdf.
- 110 For example: "Cybersecurity Preparedness for Schools and Institutions of Higher Education," U.S. Department of Education, Readiness and Emergency Management for Schools Technical Assistance Center, 2024, https://rems.ed.gov/Cyber.
- 111 U.S. Government Accountability Office, Critical Infrastructure Protection: Additional Federal Coordination Is Needed to Enhance K–12 Cybersecurity (2022), https://www.gao.gov/products/gao-23-105480.
- 112 Emsisoft, The State of Ransomware in the U.S.: Report and Statistics 2023 (2024), https://www.emsisoft.com/en/blog/44987/the-state-of-ransomware-in-the-u-s-report-and-statistics-2023/.
- 113 Sophos, The State of Ransomware in Education 2023.
- 114 "Consultancy Protocol for Building AI Capacity in Your School District," EDSAFE AI Alliance, https://drive.google.com/file/d/1-u7uq0dvSB7IddXR2hVv-KTezpCK_ic_/view.
- 115 U.S. Global Change Research Program, Fifth National Climate Assessment (2024), https://nca2023.globalchange.gov/.
- 116 Joseph B. Keller, Manann Donoghoe, and Andre M. Perry, The US Must Balance Climate Justice Challenges in the Era of Artificial Intelligence, Brookings Institution (2024), https://www.brookings.edu/articles/the-us-must-balance-climate-justice-challenges-in-the-era-of-artificial-intelligence/.
- 117 Keller, Donoghoe, and Perry, The US Must Balance Climate Justice Challenges in the Era of Artificial Intelligence.
- 118 Keller, Donoghoe, and Perry, The US Must Balance Climate Justice Challenges in the Era of Artificial Intelligence.
- 119 Niklas Sundberg, "Tackling AI’s Climate Change Problem," MIT Sloan Management Review, 2024, https://sloanreview.mit.edu/article/tackling-ais-climate-change-problem/.
- 120 Melissa Heikkilä, "Making an Image with Generative AI Uses as Much Energy as Charging Your Phone," Technology Review (Dec. 1, 2023). https://www.technologyreview.com/2023/12/01/1084189/making-an-image-with-generative-ai-uses-as-much-energy-as-charging-your-phone/.
- 121 Karen Hao, "Training a Single AI Model Can Emit as Much Carbon as Five Cars in their Lifetimes," MIT Technology Review (June 6, 2019). https://www.technologyreview.com/2019/06/06/239031/training-a-single-ai-model-can-emit-as-much-carbon-as-five-cars-in-their-lifetimes/.
- 122 Md Abu Bakar Siddik, Arman Shehabi, and Landon Marston, "The Environmental Footprint of Data Centers in the United States," Environmental Research Letters 16 (2021), https://doi.org/10.1088/1748-9326/abfba1.
D. Principle 4: Equitable access to and use of AI tools is ensured
1. Text of the Principle
Gaps in educational opportunities, resources, and funding negatively affect student outcomes and are exacerbated for students living in rural areas, those who are Native, Asian, Black, Latin(o/a/x), Middle Eastern and North African, Multiracial, or Pacific Islander, and those who are LGBTQ+. This has become clear regarding educational technology, an area where students and educators in under-resourced schools and institutions have struggled to achieve equity. Deploying AI tools will further widen this digital divide if measures are not taken to guarantee access to all students and educators, from early childhood to higher education, regardless of ZIP code. Education systems must not only provide AI tools but also guarantee the technical support, devices, and internet infrastructure necessary to reliably access and use AI in the classroom and at home.
Artificial intelligence must also be used in equitable ways in schools and on campuses. To ensure all students – regardless of race/ethnicity, disability status, emergent multilingual learner status, or location – have access to learning opportunities that use AI to promote active learning, critical thinking, and creative engagement, we have to be intentional and proactive to prevent our biases from impacting how students experience AI technology. Educators must be cognizant of the potential for some students, particularly high-need learners, including students with disabilities and emergent multilingual learners, to be relegated to using AI only for rote memorization, standardized assessment, or finding answers to factual questions. Policies and procedures must be in place to guarantee that all students—not only the most advantaged or most advanced—are able to take full advantage of AI technology.
2. Connections to Existing NEA Policies
This principle closely relates to NEA’s Policy Statement on Digital Learning. Specifically, the digital learning statement calls for equitable access to digital technologies, technical support, and infrastructure to close the achievement and digital divide while ensuring that classrooms function properly and reliably for both educators and students. Additionally, the proposed Policy Statement relates to Resolution A-14: Financial Support of Public Education, which supports that every state should ensure adequate and equitable funding to meet the needs of all students. Resolution B-36: Education for All Students with Disabilities states that a fully accessible educational environment, using appropriate instructional materials, must match the learning needs of both students with and students without disabilities. Resolution B-67: Fair and Equitable Access to Technology states that students must have access to and instruction in technology and encourages the responsible use of technology. Furthermore, the Resolution states that students should have equitable access to training, funding, and participation to ensure their technological literacy regardless of geographic, economic, social, or cultural constraints. The Task Force proposes that the same standards outlined in the above statement and resolutions should be applied to AI technologies to ensure equitable and inclusive access to AI tools and resources.
3. Background Research and Information
The Task Force believes that equitable and inclusive access to AI technologies must be a priority for educators and public schools. Research shows that divides in educational opportunities, resources, and funding can negatively affect student outcomes. For an overview of research on this topic, see C. Kirabo Jackson and Claire Mackevicius, "The Distribution of School Spending Impacts," NBER Working Papers No. 28517 (2021), https://doi.org/10.3386/w28517. Go to reference To ensure that the emergence of AI in education does not exacerbate these gaps, the proposed Policy Statement asserts that all students and educators from Pre-K through higher education should have access to AI tools and resources. Additionally, the proposed Policy Statement calls for the technical support and infrastructure necessary to reliably access and use AI in the classroom and at home. Adequate funding and support are especially needed for under-resourced schools and districts in rural, urban, and tribal areas.
The COVID-19 pandemic highlighted our nation’s significant digital divides. While some schools and higher education institutions were able to pivot quickly to virtual learning by providing students and educators with modern devices, internet hotspots, and the necessary software, others struggled, with students trying to attend virtual school using mobile phones in parking lots so that they could access the internet in nearby areas. The emergence of AI in education may widen these already significant gaps. The U.S. Department of Education's 2024 National Educational Technology Plan defines three different digital divides:
- Digital Use Divide: Inequitable implementation of instructional tasks supported by technology, with some students using technology actively—to analyze, build, produce, and create—and others using it for passive assignment completion.
- Digital Design Divide: Inequitable access to time and support of professional learning for educators to build their capacity to design learning experiences for all students using ed-tech.
- Digital Access Divide: Inequitable access to connectivity, devices, and digital content. Adapted from: U.S. Department of Education, Office of Educational Technology, A Call to Action for Closing the Digital Access, Design, and Use Divides: 2024 National Educational Technology Plan (2024), https://tech.ed.gov/netp/. Go to reference
The last divide, digital access, is the one most often thought of when the ‘digital divide’ is mentioned. While some students and educators have access to the latest devices and high-speed internet, others, particularly those in rural and under-resourced communities, are left using outdated equipment and software without consistent access to the internet. National Education Association, Digital Equity for Students and Educators (2020), https://www.nea.org/sites/default/files/2020-10/NEA%20Report%20-%20Digital%20Equity%20for%20Students%20and%20Educators_0.pdf. Go to reference Digital divides may exist within schools and higher education institutions too—some educators, particularly ESPs, may find they are asked to share devices or use equipment deemed too out of date for other educators to use.
However, the other two divides mentioned are equally important. The second divide, digital design, will be discussed in more depth in Section V.E.3. The first divide, digital use, warrants considerably more attention than it typically receives. Even if students and educators have access to AI technology, the ways in which they use it may differ greatly. For example, students in an advanced class or in a socioeconomically advantaged district may use AI to enhance their learning by creating their own movies, designing their own chatbots, or delving into rich AI tools being used to support scientific research. In contrast, less-advantaged students are more likely to encounter AI in ways that replicate rote learning that is not driven by technology, such as point-and-click tutoring systems, or that involve them passively consuming AI-generated content.
The National Educational Technology Plan provides guidance on how to close this divide, including developing learner profiles that outline competencies students should have, designing systems that help students use technology to achieve those competencies, creating opportunities for students to become co-designers of their learning experiences, and implementing Universal Design for Learning (UDL) Guidelines to ensure access for learners with disabilities. U.S. Department of Education, A Call to Action for Closing the Digital Access, Design, and Use Divides: 2024 National Educational Technology Plan. Go to reference
UNESCO’s guidance for generative AI in education speaks to the critical importance of inclusion and accessibility of generative AI. “[Generative AI] tools will not help address the fundamental challenges in education ... unless such tools are made inclusively accessible (irrespective of gender, ethnicity, special education needs, socioeconomic status, geographic location, displacement status and so on), and if they do not by design advance equity, linguistic diversities, and cultural pluralism.” The guidance recommends the following policy measures to promote inclusion, equity, and linguistic and cultural diversity:
- “Identify those who do not have or cannot afford internet connectivity or data and take action to promote universal connectivity and digital competencies in order to reduce the barriers to equitable and inclusive access to AI applications. Establish sustainable funding mechanisms for the development and provision of AI-enabled tools for learners who have disabilities or special needs. Promote the use of [generative AI] to support lifelong learners of all ages, locations, and backgrounds;
- Develop criteria for the validation of [generative AI] systems to ensure that there is no gender bias, discrimination against marginalized groups, or hate speech embedded in data or algorithms; and
- Develop and implement inclusive specifications for [generative AI] systems and implement institutional measures to protect linguistic and cultural diversities when deploying [generative AI] in education and research at scale. Relevant specifications should require providers of [generative AI] to include data in multiple languages, especially local or indigenous languages, in the training of GPT models to improve [generative AI’s] ability to respond to and generate multilingual text. Specifications and institutional measures should strictly prevent AI providers from any intentional or unintentional removal of minority languages or discrimination against speakers of indigenous languages, and require providers to stop systems promoting dominant languages or cultural norms.” Fengchun Miao and Wayne Holmes, Guidance for Generative AI in Education and Research, UNESCO (2023), https://www.unesco.org/en/articles/guidance-generative-ai-education-and-research. Go to reference
As AI becomes more and more ingrained in everyday life and classrooms, it is critical that the digital divide is severely reduced and eventually eliminated. Local, state, and federal policymakers need to ensure that adequate funding is distributed to districts and schools to not only provide the AI tools and resources needed to meet educator and student needs but also to guarantee the technical support and infrastructure necessary to reliably access and use AI in the classroom and at home. Adequate funding is especially needed for low-income, rural, and urban schools and districts.
Additionally, policymakers, in collaboration with educators and their unions, must develop AI guidance to help districts and schools navigate this transformative and rapidly growing technology. With such guidance and funding, educators will have the resources necessary to develop educational plans that will incorporate AI into teaching and learning across curricula. Any guidance and implementation around AI should be inclusive to all students and educators regardless of ability, identity, income level, learning style, or location. AI has the potential to enhance the quality of education, and all students and educators deserve to reap these benefits.
- 123 For an overview of research on this topic, see C. Kirabo Jackson and Claire Mackevicius, "The Distribution of School Spending Impacts," NBER Working Papers No. 28517 (2021), https://doi.org/10.3386/w28517.
- 124 Adapted from: U.S. Department of Education, Office of Educational Technology, A Call to Action for Closing the Digital Access, Design, and Use Divides: 2024 National Educational Technology Plan (2024), https://tech.ed.gov/netp/.
- 125 National Education Association, Digital Equity for Students and Educators (2020), https://www.nea.org/sites/default/files/2020-10/NEA%20Report%20-%20Digital%20Equity%20for%20Students%20and%20Educators_0.pdf.
- 126 U.S. Department of Education, A Call to Action for Closing the Digital Access, Design, and Use Divides: 2024 National Educational Technology Plan.
- 127 Fengchun Miao and Wayne Holmes, Guidance for Generative AI in Education and Research, UNESCO (2023), https://www.unesco.org/en/articles/guidance-generative-ai-education-and-research.
E. Principle 5: Ongoing education with and about AI: AI literacy and agency
1. Text of the Principle
Effective, safe, and equitable use of AI technology in education requires that students and educators become fully AI literate and develop a greater sense of agency with this technology. The use of artificial intelligence extends into countless aspects of our personal and professional lives, and AI literacy must be part of every student’s basic education and every educator’s professional preparation and development.
Artificial intelligence is a vital component of the computer sciences but extends far beyond the computer science curriculum. Curricular changes should be made to incorporate AI literacy across all subject areas and educational levels so that all students understand the benefits, risks, and effective uses of these tools. These student learning experiences should be developmentally appropriate, experiential (allowing students to engage with various forms of AI-enhanced technology), and help students think critically about using AI-enhanced technology.
Educators must be afforded high-quality, multifaceted, ongoing professional learning opportunities that help increase their AI literacy and understand what, how, and why specific AI is being used in their educational settings. Learning opportunities must be provided to educators in all positions and at all career stages. Educators must know how to use AI in ways that are pedagogically appropriate within their content areas and for all learners, including early learners, students with disabilities, and emergent multilingual learners. These learning opportunities must also help educators research and assess available evidence about effective AI uses in education; understand AI bias and know strategies for reporting and mitigating the harmful impacts of AI bias; and understand the ethical and data privacy hazards associated with AI-enabled technology and appropriate policies and standards in use by their educational institutions. Educators should be positioned to lead professional learning about the use of AI tools in educational settings.
2. Connections to Existing NEA Policies
This position resonates with existing policy statements and resolutions. Specifically, Resolution A-14: Financial Support of Public Education calls for professional learning funding for all educators. Resolution B-66: Technology in the Education Process states that technology improves the educational experience so long that all educators are provided adequate professional learning and training for the use, integration, and applications of technologies to enhance instruction. Resolutions D-16: Professional Development for Education Professionals and D-17: Professional Development for Education Support Professionals both call for continuous professional learning to achieve and maintain the highest standards of professional practice to meet the needs of all students. Lastly, the Policy Statement on Digital Learning, adopted by the 2013 Representative Assembly and amended in 2018, states that all educators should have access to relevant, high-quality, interactive professional learning in the integration of digital learning and the use of technology into their instruction and practice. The Task Force proposes that the same standards outlined in the above statement and resolutions should be applied to artificial intelligence to promote AI literacy for all educators and students.
3. Background Research and Information
With the implementation of generative AI tools, new possibilities for teaching and learning have emerged. AI has great potential to enhance education for all students from Pre–K through the postsecondary level. The proposed Policy Statement recognizes that AI literacy is vital for students and, therefore, advocates for the necessary curricular changes to incorporate artificial intelligence across all subject areas and education levels.
Furthermore, AI literacy will be needed for today’s students to fully succeed in many careers. This change has already started, with 66 percent of finance employers and 72 percent of manufacturing employers reporting on an OECD survey that they are already using AI to do tasks that employees used to do and about half saying that AI had created new tasks. OECD, The Impact of AI on the Workplace: OECD AI Surveys of Employers and Workers (2023), https://www2.oecd.org/future-of-work/aisurveysofemployersandworkers.htm. Go to reference In the same survey, about 40 percent of employers said that a lack of relevant employee skills was a barrier to AI adoption. Students who understand AI, when to use it, and when not to use it will undoubtedly have an edge in the workforce. Artificial intelligence that supports workers with disabilities may also open access to new career pathways for these individuals.
Artificial intelligence is already outperforming many humans on tests of adult numeracy and literacy. OECD, Is Education Losing the Race with Technology? AI's Progress in Maths and Reading (2023), https://www.oecd-ilibrary.org/education/is-education-losing-the-race-with-technology_73105f99-en. Go to reference As with past significant technological advances, it is likely that some skills will lessen in importance and occupations will dwindle or disappear as AI evolves and becomes more and more widely used. These developments underscore the NEA‘s decades-long concerns related to the U.S.’s overreliance on standardized assessments, which, for many reasons, have resulted in narrowing educational opportunities, penalizing our schools, and discouraging innovation.
While students need to learn about and with AI, they must also develop their skills in areas that AI cannot replace. Harvard Business Review provides a simple construct for breaking down these irreplaceable human qualities: 1. Curiosity, 2. Humanity, and 3. Emotional Intelligence. Tomas Chamorro-Premuzic and Reece Akhtar, "3 Human Super Talents AI Will Not Replace," Harvard Business Review, May 28, 2023, https://hbr.org/2023/05/3-human-super-talents-ai-will-not-replace. Go to reference Keeping these characteristics at the forefront of education policy, instructional design, and educational opportunities will help students better prepare for the future.
Fortunately, these qualities are also highly valued in education occupations. However, educators must become AI literate if they are to foster these qualities in their students and successfully advocate for AI to be used in line with the principles outlined in this report. When asked why they were not yet using AI tools in instruction, one of the reasons teachers most often cited—after having other priorities—is that they simply don’t know how to use them. Lauraine Langreo, "Most Teachers Are Not Using AI. Here’s Why," Education Week, January 8, 2024, https://www.edweek.org/technology/most-teachers-are-not-using-ai-heres-why/2024/01. Go to reference Educators are eager for high-quality opportunities that will help them be better at their work and better advocates for their students and their schools and campuses.
The Task Force believes that training and professional learning opportunities are crucial for promoting AI literacy among educators. Aspiring educators from traditional and non-traditional educator preparation programs will need formal training and experience with AI. Likewise, continuous professional learning opportunities should be provided for all educators—administrators, teachers, ESPs, SISP, and higher education faculty and staff—to develop their understanding and effective use of AI in the classroom and for administrative work. Training and professional learning opportunities should be evidence-based, focus on AI literacy, and be provided to educators at all levels and in all positions, with specific attention to ethical issues and risks, teaching and learning strategies across all subject areas, and using AI with students with disabilities and emergent multilingual learners.
The 2024 National Educational Technology Plan defines digital citizenship as “appropriate, responsible behavior when using technology.” Further, the plan says, “It encompasses knowledge, skills, and attitudes required to navigate the digital world respectfully and responsibly. Good digital citizens engage positively and constructively in online communities and possess good digital literacy and critical thinking skills.” U.S. Department of Education, A Call to Action for Closing the Digital Access, Design, and Use Divides: 2024 National Educational Technology Plan. Go to reference
The National Educational Technology Plan highlights five key elements of good digital citizenship, which are:
- Responsible Online Behavior, including the importance of being respectful and kind and being mindful of the impact of one’s words in digital spaces.
- Managing One’s Digital Footprint, including being mindful of one’s own digital presence and the potential impact of online actions on one’s reputation.
- Media Literacy, including the skills associated with using technology to find, evaluate, organize, create, and communicate information.
- Understanding Copyright and Intellectual Property, including respect for intellectual property and encouraging proper citation and attribution.
- Algorithmic Literacy, including the knowledge of underlying principles, processes, and biases that shape algorithms and their implications for individuals and society. U.S. Department of Education, A Call to Action for Closing the Digital Access, Design, and Use Divides: 2024 National Educational Technology Plan. Go to reference
Digital citizenship encompasses many skills and attitudes, including, but not limited to, digital literacy and AI literacy, ethical use of technology, privacy awareness, critical thinking and information literacy, advocacy for accessibility, and active and positive participation and engagement. Effective digital citizenship requires an ongoing commitment to learning, ethical engagement, and the promotion of a digital environment that is safe, inclusive, and beneficial for all. It also requires concerted efforts to provide students and educators with the resources they need to understand technology and be critical users of it, as we describe in further detail below.
a. AI Literacy and Digital Citizenship for Students
According to the World Economic Forum’s The Future of Jobs Report 2023, World Economic Forum, The Future of Jobs Report 2023 (2023), https://www.weforum.org/publications/the-future-of-jobs-report-2023/. Go to reference nearly 75 percent of companies plan to adopt AI technologies. Being a digital citizen in the age of AI involves a nuanced understanding and engagement with the digital world—one where AI technologies play a central role in shaping student and educator experiences, interactions, and opportunities. Building this understanding into pre-K–12 and higher education will help students develop into adults who can fully participate in the future workforce.
As the role of technology in society continues to grow, it is crucial that educators foster ethical AI use and digital citizenship. This includes educating students about the ethical implications of AI—including biases, privacy concerns, and algorithmic fairness—and teaching digital citizenship skills, emphasizing responsible and ethical use of AI technologies. ASCD et al., Bringing AI to School: Tips for School Leaders. Go to reference AI4K12, a joint project of the Association for the Advancement of Artificial Intelligence and the Computer Science Teachers Association, provides a useful framework for AI literacy with its “5 Big Ideas in Artificial Intelligence.”
The "5 Big Ideas” are aimed at helping students understand both how AI works and its societal impacts—both positive and negative. AI4K12 provides a range of resources for K–12 educators to use with students to develop their AI literacy. For an alternative construct see: Farhana Faruqe, Ryan Watkins, and Larry Medsker, "Competency Model Approach to AI Literacy: Research-Based Path From Initial Framework to Model," Advances in Artificial Intelligence and Machine Learning 2, no. 4 (2022), https://www.oajaiml.com/uploads/archivepdf/19411140.pdf. Go to reference Along the same lines, the International Society for Technology in Education (ISTE) provides the ISTE Standards for Students, including specific standards related to digital citizenship, "ISTE Standards for Students," ISTE, 2024, https://iste.org/standards/students. Go to reference and Digital Promise has created an AI Literacy Framework for Learners and Educators. Kelly Mills, Pati Ruiz, and Keun-woo Lee, "Revealing an AI Literacy Framework for Learners and Educators," Digital Promise, 2024, https://digitalpromise.org/2024/02/21/revealing-an-ai-literacy-framework-for-learners-and-educators/. Go to reference
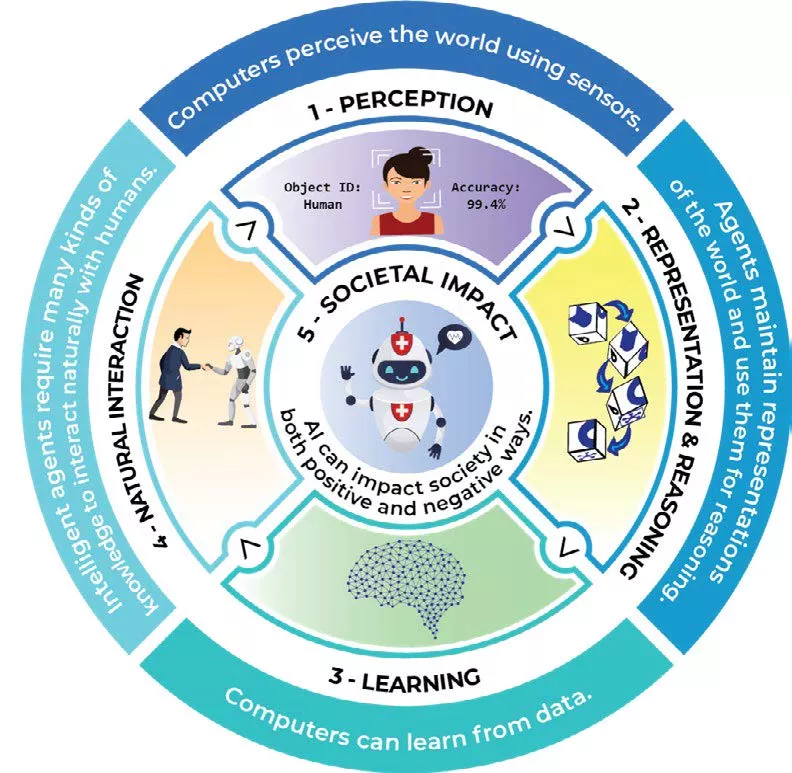
While it may feel natural to include AI literacy and digital citizenship content in computer science or other STEM courses, it is important that these skills are built throughout the curriculum. Sang Joon Lee and Kyungbin Kwon, "A Systematic Review of AI Education in K–12 Classrooms from 2018 to 2023: Topics, Strategies, and Learning Outcomes," Computers and Education: Artificial Intelligence 6 (2024), https://www.sciencedirect.com/science/article/pii/S2666920X24000122#bib21. Go to reference Artificial intelligence can also be used to help with writing, developing artwork, scanning historical documents, and foreign language translation, among other non-STEM topics. As with the internet, students need to see AI as a tool that has a role to play across the curriculum.
In addition, schools must involve families in conversations around digital literacy and citizenship. It’s important to note that not all students’ families will have high levels of digital literacy skills and, thus, may not know how to teach their children. This can lead some students to be at higher risk of engaging in inappropriate behavior online. As stated in the National Educational Technology Plan, “By approaching digital health, safety, and citizenship education holistically and engaging families as partners, school districts can build the capacity of both families and students to use technology wisely. Bringing families into the conversation about digital health, safety, and citizenship can support students while building the school-family relationships critical for academic success.” U.S. Department of Education, A Call to Action for Closing the Digital Access, Design, and Use Divides: 2024 National Educational Technology Plan. Go to reference
Several states, districts, and higher education institutions have already started considering how to ensure their students are given the AI literacy and digital citizenship skills they need. For example, the Virginia Department of Education’s guidelines suggest integrating digital citizenship within various learning strands, including computer science, digital learning, English, fine arts, health, mathematics, science, social studies, and world languages. Commonwealth of Virginia, Guidelines for AI Integration throughout Education in the Commonwealth of Virginia (2024), https://www.education.virginia.gov/media/governorvirginiagov/secretary-of-education/pdf/AI-Education-Guidelines.pdf. Go to reference Similarly, in Delaware, the legislature passed a law—the Digital Citizenship Education Act—that allows media literacy to be incorporated into existing curricula standards and states that media literacy curricula are needed to guarantee the vitality of American democracy and students’ ability to engage in civic life. "The Digital Citizenship Education Act," Delaware General Assembly, 2022, https://legis.delaware.gov/BillDetail/78981. Go to reference
The Oregon Department of Education’s guidance highlights the importance of digital literacy and citizenship among students. “Develop strong policies that include when and how generative AI can be used in the classroom,” the Department encourages its educators. “Be sure to discuss the potential risks of using AI with students (e.g., inaccurate information, bias, etc.) and provide students with digital literacy and citizenship so that they understand these risks.” The state also encourages educators to take advantage of materials that already exist. “Ensure that students understand how to use AI responsibly, ethically, and productively by integrating digital citizenship lessons into the curriculum.” Oregon Department of Education, Generative Artificial Intelligence (AI) in K–12 Classrooms (2023), https://www.oregon.gov/ode/educator-resources/teachingcontent/Documents/ODE_Generative_Artificial_Intelligence_(AI)_in_K-12_Classrooms_2023.pdf. Go to reference
While AI literacy standards and resources are widely available for pre-K–12 students, they are only just starting to emerge in higher education. In a review of existing research on AI literacy for higher and adult education, researchers find that while higher education is lagging pre-K–12 in AI literacy, there has been a notable uptick in the past few years. In addition, efforts are starting to be made to move AI literacy beyond STEM courses and professional tracks such as healthcare. Mattias Carl Laupichler et al., "Artificial Intelligence Literacy in Higher and Adult Education: A Scoping Literature Review," Computers and Education: Artificial Intelligence 3 (2022), https://doi.org/10.1016/j.caeai.2022.100101. Go to reference As with pre-K–12 education, AI needs to be included throughout the curriculum to prepare all students for full participation in society.
At the University of Florida (UF), the “AI Across the Curriculum” initiative offers AI courses in all 16 colleges, including an introductory course and a nine-course certificate program so that all students can become AI literate. "Building an AI University," University of Florida, 2024, https://ai.ufl.edu/about/. Go to reference This initiative intentionally focuses beyond STEM disciplines to broaden all students’ workforce readiness. As a group of UF faculty wrote, “AI is not simply a set of tools that can be considered in isolation, as technologies often are. AI, instead, is a comprehensive set of skills or approaches for transdisciplinary inquiry, and it encompasses, or should encompass, the full life experience and education of a learner." Jane Southworth et al., "Developing a Model for AI across the Curriculum: Transforming the Higher Education Landscape via Innovation in AI Literacy," Computers and Education: Artificial Intelligence 4 (2023), https://doi.org/10.1016/j.caeai.2023.100127. Go to reference
As technology and the power of AI continue to grow, it is critical that educators foster these skills among their students to ensure they are informed, responsible, and respectful digital citizens in an increasingly connected world.
b. AI Literacy and Digital Citizenship for Educators
Of course, educators cannot prepare students to be AI-literate digital citizens if they do not possess these skills and knowledge themselves. Educators need to model digital citizenship for students by critically evaluating online resources, engaging in civil discourse online, and using digital tools to contribute to positive social change as well as cultivating responsible online behavior, including the safe, ethical, and legal use of technology. "Artificial Intelligence," Wayne County Regional Educational Service Agency, 2024, https://www.resa.net/teaching-learning/instructional-technology/ai. Go to reference
Although we have never truly met the need for professional learning about educational technology, it is imperative that we do so now, given the lightning speed at which generative AI has blossomed. Educators must use their voices to advocate for high-quality professional learning that is accessible, equitable, job-embedded, and ongoing. There is great potential for AI to improve our education systems; however, this potential will never be realized if educators are unaware of the possibilities or lack the necessary tools and expertise to incorporate AI into their teaching practices effectively.
Implementing AI effectively and equitably involves professional learning that not only introduces educators to AI concepts and technologies but also demonstrates practical strategies for integrating AI into diverse subject areas and instructional contexts. Olivia Rütti-Joy, Georg Winder, and Horst Biedermann, "Building AI Literacy for Sustainable Teacher Education," Journal for Higher Education Development 18, no. 4 (2023), https://www.zfhe.at/index.php/zfhe/article/view/1848. Go to reference According to the Learning Forward Standards for Professional Learning, Learning Forward, Standards For Professional Learning (2022), https://learningforward.org/lf_resource/standards-for-professional-learning/. Go to reference professional learning must be rigorous for each learner; lead to improved student outcomes; sustain significant changes in knowledge, skills, practices, and mindsets; and be grounded in equity, collaboration, and educator leadership. A comprehensive AI professional learning program should be grounded in adult learning theory and include the following:
- Foundations of AI: Start with an overview of AI principles, history, and AI technologies, such as machine learning, natural language processing, and computer vision.
- Pedagogical Strategies: Show educators how to effectively incorporate AI tools and resources into teaching practices and share strategies such as how to design AI-enhanced lessons, create personalized learning experiences, and utilize AI for assessment and feedback.
- Intentional Use of AI in the Classroom: Educators are the experts when it comes to teaching and learning, so they need to use a critical eye and be intentional when incorporating AI into their teaching practices. Educators must be able to distinguish between situations where AI can enhance learning outcomes and those where its use may not be appropriate. They must also understand how AI works, have deep content knowledge of any subjects they are teaching, and have the pedagogical understanding to vet any AI-generated content or use.
- Ethical Considerations: Provide guidance on navigating the ethical implications of using AI in education. This should include privacy concerns, bias in AI systems, proprietary rights, and the impact of AI on student data security and privacy.
- Practical Applications: Offer hands-on experience with relevant AI tools. Workshops should give educators time to practice and explore AI for grading practices, use AI-powered educational games and simulations, and provide collaborative opportunities for educators to explore and discuss ways to leverage AI tools to improve teaching and learning.
- Critical Thinking and Problem-Solving with AI: Training should show educators how to foster students' AI literacy skills, such as how to assess AI tools, discern facts from misinformation, understand algorithmic bias, and consider the societal impacts of AI technologies.
- Collaborative and Project-Based Learning: Offer opportunities to explore ideas on how to integrate AI into real-world project-based learning scenarios that encourage collaboration among students.
- Ongoing Professional Learning: Professional learning should include provisions for continuous learning and regular updates on the latest AI advancements, tools, and educational applications.
- Community Building and Sharing Best Practices: Part of ongoing professional learning may include the creation of networked improvement communities (NICs) For more about NICs and improvement science, see "Improvement in Education," Carnegie Foundation for the Advancement of Teaching, accessed April 21, 2024, https://www.carnegiefoundation.org/our-work/improvement-in-education/. Go to reference where educators can share insights, challenges, and success stories about how they integrate AI into their teaching.
The National Educational Technology Plan offers one example of how a school district, Wichita (Kansas) Public Schools, has built educators’ AI literacy effectively. Example drawn from: U.S. Department of Education, A Call to Action for Closing the Digital Access, Design, and Use Divides: 2024 National Educational Technology Plan. Go to reference Leaders sought to build digital citizenship into the learning of every student. To that end, they developed a three-year plan that focused on middle schools in the first year, elementary schools in the second year, and high schools in the third. The district developed common teaching strategies and provided professional learning for teachers to build their capacity. The team leading the charge included the district’s chief information officer, digital literacy coordinator, and 12 instructional learning coaches/primary digital citizenship coaches. The core team met monthly to share new resources, provide professional learning, share best practices, address challenges, and offer collaborative support. Keys to Wichita’s success include identifying expert teachers to lead professional learning and offering insights and alignment to state standards and initiatives, such as computer science, social-emotional learning, computer literacy, and media literacy.
While this example comes from K–12 education, these learning opportunities and ongoing supports must be provided to all educators, not only K–12 teachers. It should go without saying that people preparing to be teachers need to have AI literacy content included throughout their coursework so that they may enter the profession ready to use AI safely and effectively. Furthermore, education support professionals, including K–12 paraeducators and graduate teaching assistants in higher education, often do not have consistent access to employer-provided devices, let alone the professional support needed to take full advantage of modern technology. At some higher education institutions, a focus on research rather than teaching means that faculty—and contingent faculty in particular—do not receive opportunities to hone their instructional skills and work with colleagues to develop strategies for incorporating AI into their courses. Librarians and media specialists at both pre-K–12 schools and higher education institutions need training in how to use AI in their work and how to help students navigate this new technology. Finally, specialized instructional support personnel (SISP), such as school psychologists, counselors, social workers, occupational therapists, speech therapists, and more, must become critical and skilled users of AI tools that support students with disabilities and students with mental health needs, among other considerations. None of these educators can be left out or left behind as AI literacy plans are developed and enacted. For an overview of how to implement effective professional development for teachers about digital learning, see: "Digital Learning Playbook: Providing Professional Development for Teachers," Digital Promise, accessed April 20, 2024, https://digitalpromise.org/online-learning/digital-learning-playbook/providing-professional-development-for-teachers/. Go to reference
- 128 OECD, The Impact of AI on the Workplace: OECD AI Surveys of Employers and Workers (2023), https://www2.oecd.org/future-of-work/aisurveysofemployersandworkers.htm.
- 129 OECD, Is Education Losing the Race with Technology? AI's Progress in Maths and Reading (2023), https://www.oecd-ilibrary.org/education/is-education-losing-the-race-with-technology_73105f99-en.
- 130 Tomas Chamorro-Premuzic and Reece Akhtar, "3 Human Super Talents AI Will Not Replace," Harvard Business Review, May 28, 2023, https://hbr.org/2023/05/3-human-super-talents-ai-will-not-replace.
- 131 Lauraine Langreo, "Most Teachers Are Not Using AI. Here’s Why," Education Week, January 8, 2024, https://www.edweek.org/technology/most-teachers-are-not-using-ai-heres-why/2024/01.
- 132 U.S. Department of Education, A Call to Action for Closing the Digital Access, Design, and Use Divides: 2024 National Educational Technology Plan.
- 133 U.S. Department of Education, A Call to Action for Closing the Digital Access, Design, and Use Divides: 2024 National Educational Technology Plan.
- 134 World Economic Forum, The Future of Jobs Report 2023 (2023), https://www.weforum.org/publications/the-future-of-jobs-report-2023/.
- 135 ASCD et al., Bringing AI to School: Tips for School Leaders.
- 136 For an alternative construct see: Farhana Faruqe, Ryan Watkins, and Larry Medsker, "Competency Model Approach to AI Literacy: Research-Based Path From Initial Framework to Model," Advances in Artificial Intelligence and Machine Learning 2, no. 4 (2022), https://www.oajaiml.com/uploads/archivepdf/19411140.pdf.
- 137 "ISTE Standards for Students," ISTE, 2024, https://iste.org/standards/students.
- 138 Kelly Mills, Pati Ruiz, and Keun-woo Lee, "Revealing an AI Literacy Framework for Learners and Educators," Digital Promise, 2024, https://digitalpromise.org/2024/02/21/revealing-an-ai-literacy-framework-for-learners-and-educators/.
- 139 Sang Joon Lee and Kyungbin Kwon, "A Systematic Review of AI Education in K–12 Classrooms from 2018 to 2023: Topics, Strategies, and Learning Outcomes," Computers and Education: Artificial Intelligence 6 (2024), https://www.sciencedirect.com/science/article/pii/S2666920X24000122#bib21.
- 140 U.S. Department of Education, A Call to Action for Closing the Digital Access, Design, and Use Divides: 2024 National Educational Technology Plan.
- 141 Commonwealth of Virginia, Guidelines for AI Integration throughout Education in the Commonwealth of Virginia (2024), https://www.education.virginia.gov/media/governorvirginiagov/secretary-of-education/pdf/AI-Education-Guidelines.pdf.
- 142 "The Digital Citizenship Education Act," Delaware General Assembly, 2022, https://legis.delaware.gov/BillDetail/78981.
- 143 Oregon Department of Education, Generative Artificial Intelligence (AI) in K–12 Classrooms (2023), https://www.oregon.gov/ode/educator-resources/teachingcontent/Documents/ODE_Generative_Artificial_Intelligence_(AI)_in_K-12_Classrooms_2023.pdf.
- 144 Mattias Carl Laupichler et al., "Artificial Intelligence Literacy in Higher and Adult Education: A Scoping Literature Review," Computers and Education: Artificial Intelligence 3 (2022), https://doi.org/10.1016/j.caeai.2022.100101.
- 145 "Building an AI University," University of Florida, 2024, https://ai.ufl.edu/about/.
- 146 Jane Southworth et al., "Developing a Model for AI across the Curriculum: Transforming the Higher Education Landscape via Innovation in AI Literacy," Computers and Education: Artificial Intelligence 4 (2023), https://doi.org/10.1016/j.caeai.2023.100127.
- 147 "Artificial Intelligence," Wayne County Regional Educational Service Agency, 2024, https://www.resa.net/teaching-learning/instructional-technology/ai.
- 148 Olivia Rütti-Joy, Georg Winder, and Horst Biedermann, "Building AI Literacy for Sustainable Teacher Education," Journal for Higher Education Development 18, no. 4 (2023), https://www.zfhe.at/index.php/zfhe/article/view/1848.
- 149 Learning Forward, Standards For Professional Learning (2022), https://learningforward.org/lf_resource/standards-for-professional-learning/.
- 150 For more about NICs and improvement science, see "Improvement in Education," Carnegie Foundation for the Advancement of Teaching, accessed April 21, 2024, https://www.carnegiefoundation.org/our-work/improvement-in-education/.
- 151 Example drawn from: U.S. Department of Education, A Call to Action for Closing the Digital Access, Design, and Use Divides: 2024 National Educational Technology Plan.
- 152 For an overview of how to implement effective professional development for teachers about digital learning, see: "Digital Learning Playbook: Providing Professional Development for Teachers," Digital Promise, accessed April 20, 2024, https://digitalpromise.org/online-learning/digital-learning-playbook/providing-professional-development-for-teachers/.